Latency time的問題,透過圖書和論文來找解法和答案更準確安心。 我們找到下列懶人包和總整理
Latency time的問題,我們搜遍了碩博士論文和台灣出版的書籍,推薦寫的 Network and Parallel Computing: 18th IFIP WG 10.3 International Conference, NPC 2021, Paris, France, November 3-5, 2021, Proceed 和Akidau, Tyler,Chernyak, Slava,Lax, Reuven的 Stream Processing Pocket Reference: Real-Time Any-Scale Data Processing都 可以從中找到所需的評價。
另外網站Who moved my 99th percentile latency? | LinkedIn Engineering也說明:Longtail latencies affect members every day and improving the response times of systems even at the 99th percentile is critical to the ...
這兩本書分別來自 和所出版 。
國立陽明交通大學 電子研究所 侯拓宏所指導 葉淑銘的 應用於脈衝神經元之閥門開關選擇器: 元件特性分析與模型開發 (2021),提出Latency time關鍵因素是什麼,來自於脈衝神經元、閾值開關選擇器、模型開發。
而第二篇論文國立陽明交通大學 智慧計算與科技研究所 謝君偉所指導 周承翰的 平均位移法應用於可微分式架構搜索 (2021),提出因為有 電腦視覺、深度學習、影像辨識、神經架構搜索、可微分式架構搜索的重點而找出了 Latency time的解答。
最後網站What is Latency in Networking? - Apposite Technologies則補充:As network engineers, when we say latency, we're generally referring to the amount of time it takes to send a packet of data from one location to another. Users ...
Network and Parallel Computing: 18th IFIP WG 10.3 International Conference, NPC 2021, Paris, France, November 3-5, 2021, Proceed
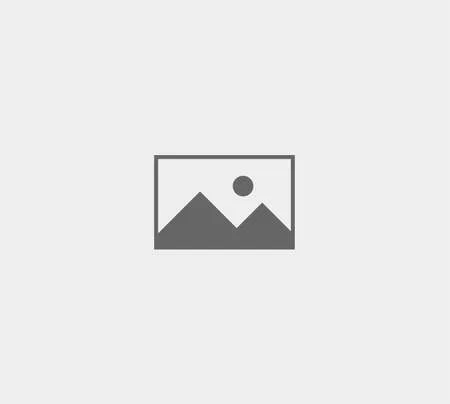
為了解決Latency time 的問題,作者 這樣論述:
Algorithms and Applications.- High Resolution of City-Level Climate Simulation by GPU with Multi-Physical Phenomena.- dgQuEST: Distributed-GPU Accelerated Large Scale Quantum Circuit Simulation.- vSketchDLC: A Sketch on Distributed Deep Learning Communication via Fine-grained Tracing Visualization.-
Scalable Algorithms Using Sparse Storage for Parallel Spectral Clustering on GPU.- XSP: Fast SSSP Based on Communication-Computation Collaboration.- A Class Of Fast And Accurate Multi-layer Block Summation And Dot Product Algorithms.- A KNN Query Method for Autonomous Driving Sensor Data.- System S
oftware and Resource Management.- A Novel Task-Allocation Framework based on Decision-Tree Classification Algorithm in MEC.- QoS-Aware Scheduling for Cellular Networks Using Deep Reinforcement Learning.- Adaptive Buffering Scheme for PCM/DRAM-Based Hybrid Memory Architecture.- Efficiency-First Fault
-Tolerant Replica Scheduling Strategy for Reliability Constrained Cloud Application.- Towards an Optimized Containerization of HPC Job Schedulers based on Namespaces.- Architecture of an On-time Data Transfer Framework in Cooperation with Scheduler System.- Storage.- Data Delta Based Hybrid Writes f
or Erasure-Coded Storage Systems.- BDCuckoo: An Efficient Cuckoo Hash for BlockDevice.- A Two Tier Hybrid Metadata Management mechanism for NVM Storage System.- A Novel CFLRU-based Cache Management Approach for NAND-based SSDs.- Networks and Communications.- Taming Congestion and Latency in Low-Diam
eter High-Performance Datacenters.- Evaluation of Topology-Aware All-reduce Algorithm for Dragon y Networks.- MPICC: Multi-Path INT-based Congestion Control in Datacenter Networks.
Latency time進入發燒排行的影片
การ์ดแคปเจอร์ กล่องแคปเจอร์ แม้ว่าจะเรียกปะปนกันไปมา หลายท่านก็เข้าใจว่ากำลังสื่อถึงอะไร แต่หลายท่านก็อาจจะยังสับสนว่า ตกลงแล้วมันเรียกว่าอะไรแน่? ซึ่งหากจะให้ถูกต้อง สำหรับเจ้าสิ่งที่จะมาทดสอบ มาลองใช้งานหรือรีวิวในวันนี้จากทาง EVGA นั้นเราจะต้องเรียกว่า "กล่องแคปเจอร์" เพราะมันมาเป็นกล่องหรือเชื่อมต่อใช้งานแบบภายนอกผ่าน USB ส่วนถ้าจะเรียกว่า "การ์ดแคปเจอร์" เราจะใช้เรียกตัวที่เป็นการ์ด PCI Express สำหรับเสียบตรงลงบนเมนบอร์ด แต่สำหรับหน้าที่การทำงานจะไม่แตกต่างกัน
สำหรับตรงนี้ กับการทดสอบใช้งานกล่องแคปฯ EVGA XR1 นั้น จะเป็นการลองใช้งาน ลองดูลูกเล่นต่าง ๆ ของมันว่ามีอะไรให้ปรับให้ใช้งานบ้าง ใช้ยากง่ายเพียงไร? และแน่นอนว่าจะรวมถึงความสามารถหลักคือการดักจับภาพ จะทั้งจากกล้องและจากการ์ดจอ เพื่อดูว่าภาพที่ได้นั้นจะให้สีสดใสเป็นธรรมชาติขนาดไหนในแบบค่าเดิม ต้องมาปรับแต่งเองเพิ่มไหม ให้ภาพที่ไหลลื่นดีไหม ซึ่งก็จะทำการเปรียบเทียบกับกล่องแคปจาก Razer HD ที่ใช้งานอยู่ ใครที่กำลังมองหาการ์ดแคปฯเพื่อมาใช้งานก็ลองชมและตัดสินใจกันดูนะครับ #EvgaXR1 #CaptureCard #กล่องแคปเจอร์
เปิดกล่องพรีวิว : https://youtu.be/enG7JURfKWI?t=911
EVGA XR1 Capture Device ราคา 6,590 บาท รับประกัน 2 ปี รายละเอียดเพิ่มเติม : https://bangmod.co.th/product/evga-xr1-capture-device/
Time Stamps
0:00 : เพลงเริ่มรายการ
2:59 : Start : ช่วงเริ่มรายการ แนะนำเนื้อหาในวันนี้
7:14 : เตรียมตัวทดสอบ
11:03 : Compare : เปรียบเทียบสีและ Latency ระหว่าง EVGA XR1 กับ Razer Ripsaw HD
23:54 : Compare : เปรียบเทียบสีและ Latency ระหว่าง EVGA XR1 กับ Elgato 4K (เกม PUBG)
34:07 : Mic : ทดสอบเสียงไมค์ที่ออกจาก EVGA XR1
52:46 : OBS : ทดสอบใช้งาน EVGA XR1 ผ่าน OBS
55:02 : Software : ทดลองโหลดใช้งาน Software ของ EVGA XR1
1:02:42 : พูดคุยหลังการทดสอบ
1:36:29 : Result : บทสรุปกับ EVGA XR1
1:44:47 : ช่วงท้ายรายการ
ติดตามผลงานของเรา...
ติดตามผ่าน Facebook ได้ที่ : https://www.facebook.com/ZoLKoRn
ติดตามผ่าน Twitch : https://www.twitch.tv/ZoLKoRn
ติดตามผ่าน Twitter ได้ที่ : https://twitter.com/ZoLKoRn
ติดตามเว็บไซต์ : http://www.zolkorn.com
ติดต่อเรา : http://www.zolkorn.com/contact/
พูดคุยระบบเสียง : https://www.youtube.com/channel/UCwY27ufiIhMXn_PO9hgCIjA
Credit :
___________________________
Countdown music :
Titre : Claw Through
Artist : Austin Woodward
Powered by: https://www.facebook.com/echopraxiaband/
End music :
Titre : Nothing I Won't Do (feat. Kianna)
Artist : Giulio Cercato
Powered by: https://www.facebook.com/GiulioCercatoMusic/
應用於脈衝神經元之閥門開關選擇器: 元件特性分析與模型開發
為了解決Latency time 的問題,作者葉淑銘 這樣論述:
隨著這個世代對數據存儲與處理的需求不斷增長,使用傳統馮諾依曼(von-Neumann)架構的計算系統面臨著速度上的限制。這是因爲傳統馮諾依曼架構中分離的處理器和記憶體單元之間頻繁的數據傳輸使得計算效率無法提升。近年來,受人類大腦運作模式啟發的類神經計算(brain-inspired computing)成為一個引人注目的話題。與傳統計算系統不同的是,類神經計算(neuromorphic computing)通過使用交錯式記憶體陣列(crossbar memory array)實現記憶體內計算(in-memory computing),進而縮短了數據傳輸的時間延遲。因此,類神經計算被視為非常有
潛力成為非馮諾依曼架構之候選人。為了開發具有高性能、低功耗特性的類神經計算硬體,使用元件為基礎(device-based)之人工突觸(synapse)和神經元(neuron)受到廣泛的研究。其中,利用閾值切換(threshold switching,TS)選擇器(selector)所構建之人工神經元有著比傳統以CMOS所建構之神經元電路面積小40倍的優勢,因此被認為是前景看好的候選人之一。因此,學術界提出了一個電路層級之模型來進一步研究 TS 神經元的行爲。此模型透過考慮神經元電路中的電阻電容延遲(RC delay) 來執行 TS 神經元之行為。然而,該模型並沒有考慮 TS 神經元中 TS 選
擇器的實際元件特性。因此,目前還缺乏一個有綜合考慮TS 神經元元件特性以及電路RC 延遲的模型。在本論文中,我們構建了一個以成核理論(nucleation theory)爲基礎的電壓-時間轉換模型(V-t transition model)來預測和模擬 TS 神經元的行為。據我們所知,這是第一個詳細考慮了 TS 選擇器中元件成核條件的 TS 神經元模型。模擬結果也顯示了 TS 選擇器的元件特性與 TS 神經元行為之間存在很強的相關性。最后,此V-t 模型為 TS 神經元的未來發展提供了一個良好的設計方針:即具有低 τ0 的 TS 選擇器是首選。因此,模擬結果顯示,與IMT (insulator
-metal-transition) 和Ag-based神經元相比,具有極小τ0的OTS (ovonic threshold switching) 神經元擁有最理想的特性。
Stream Processing Pocket Reference: Real-Time Any-Scale Data Processing
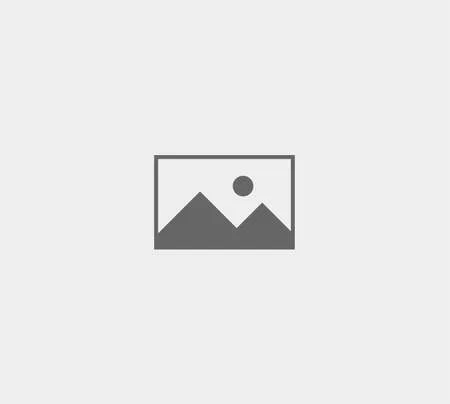
為了解決Latency time 的問題,作者Akidau, Tyler,Chernyak, Slava,Lax, Reuven 這樣論述:
Tyler Akidau is principal software engineer at Snowflake. Previously senior staff software engineer at Google, he was the technical lead for the Data Processing Languages & Systems group, responsible for Google’s Apache Beam efforts, Google Cloud Dataflow, and internal data processing tools like Goo
gle Flume, MapReduce, and MillWheel. His also a founding member of the Apache Beam PMC. Though deeply passionate and vocal about the capabilities and importance of stream processing, he is also a firm believer in batch and streaming as two sides of the same coin, with the real endgame for data proce
ssing systems the seamless merging between the two. He is the author of the 2015 Dataflow Model paper and the Streaming 101 and Streaming 102 articles on the O’Reilly website. His preferred mode of transportation is by cargo bike, with his two young daughters in tow.Slava Chernyak is a senior softwa
re engineer at Google Seattle. Slava spent over five years working on Google’s internal massive-scale streaming data processing systems and has since become involved with designing and building Windmill, Google Cloud Dataflow’s next-generation streaming backend, from the ground up. Slava is passiona
te about making massive-scale stream processing available and useful to a broader audience. When he is not working on streaming systems, Slava is out enjoying the natural beauty of the Pacific Northwest.Reuven Lax is a senior staff software engineer at Google Seattle, and has spent the past nine yea
rs helping to shape Google’s data processing and analysis strategy. For much of that time he has focused on Google’s low-latency, streaming data processing efforts, first as a long-time member and lead of the MillWheel team, and more recently founding and leading the team responsible for Windmill, t
he next-generation stream processing engine powering Google Cloud Dataflow. He’s very excited to bring Google’s data-processing experience to the world at large, and proud to have been a part of publishing both the MillWheel paper in 2013 and the Dataflow Model paper in 2015. When not at work, Reuve
n enjoys swing dancing, rock climbing, and exploring new parts of the world.Austin Bennett designs data systems to help move, share, gather insights and develop data products efficiently.
平均位移法應用於可微分式架構搜索
為了解決Latency time 的問題,作者周承翰 這樣論述:
在神經架構搜索(NAS)中,可微分式架構搜索(DARTS)是一項基於連續鬆弛網路且有效率的搜索方法,同時能在較低的搜索運算資源中實現。DARTS不僅在自動化機器學習(Auto-ML)領域中吸引了很多研究者的關注,且在近期被視為NAS領域的標竿方法之一。儘管DARTS在搜索效率上比傳統的NAS方法更佳,但DARTS在離散轉換到連續的過程卻有著搜索穩定性的問題。經過再現實驗並觀察,發現DARTS的搜索不穩定性會導致驗證階段的準確率急劇降低,為了解決此問題,此篇論文提出將平均位移(Mean-Shift)應用於DARTS上,在搜索時加入此一方法的採樣並配合搜索擾動機制。這方法在物理意義上,藉由適當的
Mean-Shift參數平滑化連續情況下搜索時的損失,來提升DARTS的搜索穩定性和驗證階段的準確率。而平均位移應用於DARTS上的收斂性,可以依據平均位移法的半徑長度等參數來做調整。最終我將此一方法驗證在諸個公開的資料集上,如:Cifar10、Cifar100、ImageNet,且在這些資料集上的準確率都達到相關方法的最佳。
想知道Latency time更多一定要看下面主題
Latency time的網路口碑排行榜
-
#1.Time-Delay Latency of Resting-State Blood Oxygen Level ...
Notably, the FC, taking into account different levels of latency or time delays between the dynamics of pairs of brain regions, results in ... 於 www.liebertpub.com -
#2.Primer on Latency and Bandwidth - High Performance ...
The time data spends queued inside the buffer is, not surprisingly, known as queuing delay. Each packet traveling over the network will incur many instances of ... 於 hpbn.co -
#3.Who moved my 99th percentile latency? | LinkedIn Engineering
Longtail latencies affect members every day and improving the response times of systems even at the 99th percentile is critical to the ... 於 engineering.linkedin.com -
#4.What is Latency in Networking? - Apposite Technologies
As network engineers, when we say latency, we're generally referring to the amount of time it takes to send a packet of data from one location to another. Users ... 於 www.apposite-tech.com -
#5.What is Data Latency? | Earthdata - NASA
Data providers use terms such as near real-time and low latency to refer to data provided to users quickly, but what do these terms mean? 於 earthdata.nasa.gov -
#6.Latency Modes | Microsoft Docs
To reclaim objects, the garbage collector (GC) must stop all the executing threads in an application. The period of time during which the ... 於 docs.microsoft.com -
#7.Reducing search indexing latency to one second - Twitter Blog
Most features in the Twitter application prioritize the newest relevant Tweets. Therefore, we sort our index by the time a Tweet was created. 於 blog.twitter.com -
#8.Low Latency: What It Is, Meaning & Definition - Informatica
... messages with minimal delay (latency). These networks are designed to support operations that require near real-time access to rapidly changing data. 於 www.informatica.com -
#9.Latency metrics | Cloud Spanner
Latency and operations per second. When an instance processes a small number of requests during a period of time, the 50th and 99th percentile latencies during ... 於 cloud.google.com -
#10.What Is Latency and How Do You Fix It? | Reviews.org
Latency, also called ping, measures how much time it takes for your computer, the internet, and everything in between, to respond to an action ... 於 www.reviews.org -
#11.latency time - 潛伏時間 - 國家教育研究院雙語詞彙
潛伏時間. latency time. 2003年6月 資訊與通信術語辭典. 名詞解釋: 同【潛伏】(latency)。 潛伏時間. latency time. 以latency time 進行詞彙精確檢索結果 ... 於 terms.naer.edu.tw -
#12.What Is Network Latency? Typical Causes & Ways to Reduce It
In other words, latency meaning in networking refers to the time it takes for the request sent from the browser to be processed and returned by ... 於 sematext.com -
#13.Expanding across time to deliver bandwidth efficiency and low ...
Datacenters need networks that support both low-latency and high-bandwidth packet delivery to meet the stringent requirements of modern applications. 於 www.usenix.org -
#14.Ping matters: Why low latency internet gives you the advantage
There are four areas where you're most likely to notice the difference in latency speeds: Online gaming, particularly in games that require fast reaction times ... 於 superloop.com -
#15.Latency and Backlog Bounds in Time-Sensitive Networking ...
Latency and Backlog Bounds in Time-Sensitive Networking with Credit Based Shapers and Asynchronous Traffic Shaping. Abstract: We compute bounds on ... 於 ieeexplore.ieee.org -
#16.Monthly IP Latency Data | Verizon Enterprise Solutions
See average monthly IP latency on core areas of the Verizon network. ... the contiguous US PIP Network and averaging the results over a thirty day period. 於 enterprise.verizon.com -
#17.What Is Latency? Latency vs. Bandwidth vs. Throughput
Latency refers to the delay that happens between when a user takes an action on a network or web application and when they get a response. 於 www.fortinet.com -
#18.Global Latency and Its Impact on Today's Real-Time World
Today's internet is no longer “good enough” to support the surging demand from real-time applications. 於 subspace.com -
#19.Experience API Latency - Dynamic Yield Knowledge Base
As an IT engineer, you may have some concerns or questions regarding DY's API latency, meaning the time it takes an API call's response... 於 support.dynamicyield.com -
#20.Evaluating Data Latency for Real-Time Databases | Rockset
RockBench is a benchmark designed to measure the data latency of a real-time database. This paper describes RockBench in detail and the results from running ... 於 rockset.com -
#21.What is Lower Latency? | Answer from SUSE Defines
A lower latency network connection is one that experiences very small delay times. Latency is the amount of time a message takes to traverse a computer ... 於 www.suse.com -
#22.a comparison of P300 latency and reaction time - PubMed
We confirm that the latency of the P300 component of the human event-related potential is determined by processes involved in stimulus evaluation and ... 於 pubmed.ncbi.nlm.nih.gov -
#23.latency time 中文 - 查查綫上辭典
latency time 中文::等待時間…,點擊查查權威綫上辭典詳細解釋latency time的中文翻譯,latency time的發音,音標,用法和例句等。 於 tw.ichacha.net -
#24.Latency and Response Time: The Same but Not The Same
It is important to differentiate between Latency and Response Time in order to properly analyze storage system I/O performance. 於 www.cirrusdata.com -
#25.Real-Time Latency: Rethinking Remote Networks - Telesat
Latency is time delay over a communications link, often measured as round trip time in milliseconds. On the Internet, user devices request data and servers ... 於 www.telesat.com -
#26.Latency - TestMy.net Internet Speed Test
What is Latency? Latency is the amount of time it takes for a computer or application to respond to your request. The less time it takes the better. 於 testmy.net -
#27.Prognostic Impact of the Latency Time from the Administration ...
Introduction: Among Therapy-Related Myeloid Neoplasms (t-MNs), there are two large groups depending on the cytotoxic trigger. 於 ashpublications.org -
#28.Low-latency time-of-flight non-line-of-sight imaging at 5 frames ...
Here, the authors introduce a multipixel time-of-flight NLOS imaging approach, combining array detectors and a fast algorithm, ... 於 www.nature.com -
#29.How to calculate packet time from latency and bandwidth
Propagation time = (Frame Serialization Time) + (Link Media Delay) + (Queueing Delay) + (Node Processing Delay - if known). Formulas:. 於 stackoverflow.com -
#30.Importance of Latency in Factory Automation (Rev. A) - Texas ...
The latency through the Ethernet Physical Layer is a key limiting factor of control cycle time in these applications. The capability of the DP83867 and ... 於 www.ti.com -
#31.Troubleshoot high latency on a DynamoDB table - Amazon AWS
I'm seeing an increase in the response time for Amazon DynamoDB requests. Resolution. When analyzing the Amazon CloudWatch metric ... 於 aws.amazon.com -
#32.What is Network Latency? | Glossary
Network latency is the total time, usually measured in milliseconds, required for a server and a client to complete a network data exchange. Read more. 於 www.a10networks.com -
#33.Make it Faster: More Throughput or Less Latency? - NI
There are two ways to time the acquisition of a measurement. The first is software timing: it requires input from the computer to control the ... 於 www.ni.com -
#34.Let's Take a Bit of Time to Talk about Low Latency - ADVA ...
Talking to customers who are interested in lowest latency solutions, the term "Bit Time" comes up over and over again. But it is not clear what it actually ... 於 www.blog.adva.com -
#35.Network Latency - Common Causes and Best Solutions | IR
Insufficient bandwidth – with a slow internet connection, insufficient bandwidth means that data packets take more time reach their destination, and often ... 於 www.ir.com -
#36.Prediction Latency — scikit-learn 1.0.2 documentation
Prediction Time per Instance - Bulk (100), 100 feats. Evolution of Prediction Time with #Features; Prediction Throughput for different estimators (100 ... 於 scikit-learn.org -
#37.Measuring Latency Time – Design Gateway's Technology Blog
In this case, we will demonstrate latency time in 2 cases which are software application and hardware application, which we are focusing on ... 於 dgway.com -
#38.What is Latency? | Twilio
Latency is the time delay between the initiation of an event and its reception. In telecommunications and networking, latency is often also called lag. 於 www.twilio.com -
#39.Behavior Assessment: Duration and Latency Recording - Activity
Record the length of time the behavior occurred. 4. Repeat the above steps until the end of the observation period. 5. Calculate the total duration by adding ... 於 iris.peabody.vanderbilt.edu -
#40.Data latency: What is truly important to know about it?
In short, data latency is the time it takes for data to travel from one place to another. Companies usually measure the latency in terms of two types of ... 於 blog.datumize.com -
#41.How to Calculate Packet Time from Latency and Bandwidth
Learn the basics of network bandwidth, latency, packet time in detail. 於 www.baeldung.com -
#42.Chapter 3. Real-Time and Low Latency - Fedora Docs
Why Low Latency Is Desirable. When computer audio specialists talk about a computer acting in real-time, they mean that it is acting with only an imperceptible ... 於 docs.fedoraproject.org -
#43.Network Latency Test Tool - Improve Response Time
The Quality of Experience (QoE) dashboard in SolarWinds ® Network Performance Monitor (NPM) is built to measure network response time and run network latency ... 於 www.solarwinds.com -
#44.How to Reduce Latency or Lag in Gaming - ScreenBeam
With higher speeds, this time reduces significantly. Make sure your WiFi connection is reliable. Most gaming enthusiasts believe you MUST have a wired ... 於 www.screenbeam.com -
#45.Response Latency - an overview | ScienceDirect Topics
Latency period is measured as the interval from the beginning of each stimulus to the beginning of each response. An abnormal latency time, defined as a value ... 於 www.sciencedirect.com -
#46.Latency Period of Mesothelioma: How Long the Cancer Takes ...
Mesothelioma Latency Period. Mesothelioma can take 20-50 years to develop after initial asbestos exposure. It is very rare to have a mesothelioma latency period ... 於 www.asbestos.com -
#47.What is Latency? - Network Meaning & Reduction Techniques
Latency is the time that elapses between a user action and the subsequent response. In networking, latency refers precisely to delays that occur ... 於 www.agora.io -
#48.Ping vs latency vs jitter - Ghost Gaming Broadband
In simple terms, latency is the reaction speed of your internet connection, i.e. the time it takes for data to travel from your device to ... 於 www.ghostgb.co.uk -
#49.What is Latency, TTFB, Bandwidth, and Throughput? - Geekflare
Now, the elephant in the room is network performance, which depends upon certain factors, to name a few – Latency, Time To First Byte (TTFB) ... 於 geekflare.com -
#50.End-to-End Latency Performance - AppDynamics ...
The response time metric for a business transaction provides performance information ... End-to-end latency metrics can reflect the response times for such ... 於 docs.appdynamics.com -
#51.latency time-翻译为中文-例句英语
使用Reverso Context: Learn how Overprovisioning can reduce access latency time, maintain high performance levels and help meet endurance and service life ... 於 context.reverso.net -
#52.延遲(工程學) - 維基百科,自由的百科全書
延遲(英語:Latency)也譯潛伏時間,它是指做出觸發動作與得到回應之間的時間間隔。延遲實際上是任何物理相互作用在有限速度內傳播產生的結果。 於 zh.wikipedia.org -
#53.PRTG monitors the latency of your network - Paessler
Network latency: Test vs. monitoring. One-time latency test. A simple ping query is all it takes to obtain ... 於 www.paessler.com -
#54.What is Latency? - WhatIs.com - TechTarget
Latency can be thought of as the time it takes to perform an action, while throughput can be thought of as the number of actions that can be executed in one ... 於 whatis.techtarget.com -
#55.Latency: What is it and why does it matter? - Everstream
Latency is the literal time it takes for a packet of data to reach its destination. The measurement related to latency is regarded in milliseconds. Just 150 ... 於 everstream.net -
#56.Understanding latency - Web Performance | MDN
Latency is the time it takes for a packet of data to travel from source to a destination. In terms of performance optimization, ... 於 developer.mozilla.org -
#57.What is Latency? - Definition from Techopedia
Latency is a networking term to describe the total time it takes a data packet to travel from one node to another. In other contexts, when a ... 於 www.techopedia.com -
#58.What is Latency? - GeeksforGeeks
Being simple latency means whenever you have given input to the system and the total time period it takes to give output so that particular ... 於 www.geeksforgeeks.org -
#59.Faster Low-Latency 5G Mobile Networks | Anritsu Taiwan
Implementing faster and lower-latency 5G mobile networks requires both eCPRI/RoE and high-accuracy latency measurements as well as time synchronization ... 於 www.anritsu.com -
#60.What Is Latency? And What Can I Do About It? - Ridge Cloud
Network Latency Definition #1: One-way Latency. For simple, one-way data transmission, latency is the time it takes to receive a data packet ... 於 www.ridge.co -
#61.Introduction to Ethernet Latency - Marvell
Latency in the context of networking is the time expended by propagation through the network medium and the adapter hardware, as well as the execution times of ... 於 jp.marvell.com -
#62.What Is Low Latency? - Cisco
At each step, tiny delays are introduced, which can add up to discernible wait times for the user. As overall network traffic continues to grow, latency is ... 於 www.cisco.com -
#63.What is latency? | How to fix latency | Cloudflare
Latency is the time it takes for data to pass from one point on a network to another. Suppose Server A in New York sends a data packet to Server B in London. 於 www.cloudflare.com -
#64.Real Time Some Notes on Microcontroller Interrupt Latency
Just to be clear, latency is usually specified as the time between the interrupt request and execution of the first instruction in the ... 於 www.digikey.com -
#65.Difference Between Message Latency and Time Accuracy
Our internal tests show latency values of around 15 ms when logging messages over COM ports. This means that the time reported in a log is accurate to 20 nano ... 於 novatel.com -
#66.Real-time monitoring of network latency in Software Defined ...
For each registered flow, controller composes individual link latencies on that path to compute end-to-end latency. We also propose another approach to measure ... 於 www.researchgate.net -
#67.網路的延遲(Latency)與頻寬(Bandwidth)是什麼?
延遲(Latency):一個封包從來源端送出後,到目的端接收到這個封包,中間所花的時間。 頻寬(Bandwidth):傳輸媒介的最大吞吐量(throughput)。 於 blog.gtwang.org -
#68.Latency messages - IBM
Latency messages provide metrics about transaction durations during processing. ... var://service/time-response-complete. Gets the value from latency ... 於 www.ibm.com -
#69.Latency - CableLabs
Network latency is the time that elapses between a request for information and its arrival. Because it takes time for a signal to pass through wire or fiber ... 於 www.cablelabs.com -
#70.latency time - 中文百科知識
latency time : 等待時間. 相關詞條. latency time. laten time: 等待時間 · average access time. average access time是指平均存取時間,通常它是硬碟average seek ... 於 www.easyatm.com.tw -
#71.definition of Latency time by The Free Dictionary
Also found in: Thesaurus, Medical, Encyclopedia. Related to Latency time: seek time. incubation period. n. (Pathology) med the time between exposure to an ... 於 www.thefreedictionary.com -
#72.Live streaming latency - YouTube Help - Google Support
Stream latency is the delay between your camera capturing an event and the event being displayed to viewers. When setting up your live stream, think about ... 於 support.google.com -
#73.What is server latency and why is it important? - ApacheBooster
The amount of time it takes for a message to travel across a system is called latency. Latency depends upon the speed of the medium that is transmitted. 於 apachebooster.com -
#74.Data availability and latency | Adobe Analytics
Some capabilities in the Adobe Experience Cloud come with an innate amount of latency on top of standard processing time. 於 experienceleague.adobe.com -
#75.What Is Latency and How to Reduce It - KeyCDN Support
In terms of network latency, this can be defined by the time it takes for a request to travel from the sender to the receiver and for the ... 於 www.keycdn.com -
#76.Relationship between network and application latency
To put into context the relationship between network delay and an application response time is far from one-to-one. The following example provides a ... 於 www.calnexsol.com -
#77.Bandwidth vs. Latency: What is the Difference?
This delay is known as input lag. Latency isn't just a gaming issue. Every time you put in a request to your internet connection (search for ... 於 www.highspeedinternet.com -
#78.What is a Response Latency? Definition & FAQs | ScyllaDB
Latency refers to the delay in the system, while response time includes both the delays and the actual processing time. There are many types of latency. Network ... 於 www.scylladb.com -
#79.A Just-In-Time Networking Framework for Minimizing Request ...
... to as just-in-time (JIT) communication, to support client-server applications with stringent request-response latency requirement. 於 arxiv.org -
#80.What is Page Load Time | Round Trip, TTFB and Latency
Reduce page load speed and discover what's behind page load time, how to reduce round trip time and reduce latency, and how to measure using RTT and TTFB. 於 www.imperva.com -
#81.Latency Period - Oxford Reference
1. The interval from initiation of the disease to clinical emergence of the disease (e.g., appearance of manifestations) or to disease detection. Thus, ... 於 www.oxfordreference.com -
#82.延遲時間Latency Time。 - 解釋頁
延遲時間Latency Time。 延遲時間指的是光學頭到達目標軌後,碟片轉到目標資料區所需花費的時間,理論平均值為轉半圈的時間。由於磁頭等待時間對系統整體性能影響相當 ... 於 www.yesfund.com.tw -
#83.Latency in optical fiber systems - CommScope
In telecommunications, latency describes the time delay of a packet traveling through a network or the delay imposed on a signal traveling in a transmission ... 於 www.commscope.com -
#84.The Latency Period as Logical Time | Cairn.info
1Lacan's text on “logical time” teaches us that “subjective assertion” is founded on anticipated certainty. As a conclusion to the logical movement of the ... 於 www.cairn.info -
#85.Latency in live network video surveillance - Axis ...
In net- work technology, latency is commonly perceived as the delay between the time a piece of information is sent from the source and the time the same piece ... 於 www.axis.com -
#86.Latency and Cybersickness: Impact, Causes, and Measures. A ...
Latency is a key characteristic inherent to any computer system. Motion-to-Photon (MTP) latency describes the time between the movement of a ... 於 www.frontiersin.org -
#87.Latency Definition & Meaning | Dictionary.com
In conditioning, the period of apparent inactivity between the time the stimulus is presented and the moment a response occurs. latency period. The American ... 於 www.dictionary.com -
#88.Campaign latency period - digital analytics definition - AT ...
Discover what "campaign latency period" means in digital analytics, and learn many other terms in our digital intelligence glossary. 於 www.atinternet.com -
#89.It's Time for Low Latency
latency for too long. In the past, speed-of-light delays in wide area networks and unoptimized network hard- ware have made sub-100is round-trip times ... 於 www.scs.stanford.edu -
#90.What is Latency? How Latency Works (with Examples)
Latency is the delay between a user's action and a web application's response to that action, also referred to as total round trip time. 於 blog.stackpath.com -
#91.Latency Definition
Latency is the amount of time a message takes to traverse a system. In a computer network, it is an expression of how much time it takes for ... 於 www.linfo.org -
#92.How do I Know if My Internet Connection is “Good?” | PingPlotter
This travel time is called latency. To be specific, latency (or ping) is the measure of how long it takes (in milliseconds) for one data packet to travel from ... 於 www.pingplotter.com -
#93.The Difference Between RAM Speed and CAS Latency - Crucial
In the history of memory technology, as speeds have increased, (which means clock cycle times have decreased), the CAS latency values have also increased, ... 於 www.crucial.com -
#94.Network Latency Guide: How to Check, Test, & Reduce
High latency can become increasingly problematic as networks grow bigger · Network latency is the time it takes for data or a request to go from ... 於 www.dnsstuff.com -
#95.一起幫忙解決難題,拯救IT 人的一天
先來了解來回通訊延遲時間(round-trip delay time). 所以要先了解什麼是網路延遲 ... 會影響網路速度的兩個重要因素就是延遲(Latency)與頻寬(Bandwidth):. 於 ithelp.ithome.com.tw -
#96.latency time - Linguee | 中英词典(更多其他语言)
大量翻译例句关于"latency time" – 英中词典以及8百万条中文译文例句搜索。 於 cn.linguee.com -
#97.系統設計入門:延遲與吞吐量 - Askie's Coding Life
說這兩個詞彙(Latency 和Throughput)常常會被搞混,有時候可以交換使用, ... Start Time:請求開始的時間; Response Time:資源開始下載的時間; End Time:請求結束 ... 於 askie.today -
#98.Latency period Definition & Meaning - Merriam-Webster
Recent Examples on the Web The latency period for lung cancer from radiation is longer than five years and 74 mSv spread over four years is not enough dose ... 於 www.merriam-webster.com