window frame的問題,透過圖書和論文來找解法和答案更準確安心。 我們找到下列懶人包和總整理
window frame的問題,我們搜遍了碩博士論文和台灣出版的書籍,推薦Rioux, Jonathan寫的 Data Analysis with Python and Pyspark 和洪錦魁的 Excel VBA最強入門邁向辦公室自動化之路王者歸來下冊(全彩印刷)都 可以從中找到所需的評價。
另外網站window frame 英文中文翻譯- 英漢汽車辭典 - 潤滑油也說明:英文: window frame. 中文: 玻璃框. 常見於汽車系統 > 701 車門及玻璃 > 70155 前擋風玻璃wind shield. 說明: image from http://www.infovisual.info.
這兩本書分別來自 和深智數位所出版 。
國立臺灣師範大學 科技應用與人力資源發展學系 李隆盛所指導 楊秀全的 範例引導與問題導向混合學習策略對國小學生機器人程式學習成效的影響 (2021),提出window frame關鍵因素是什麼,來自於範例引導與問題導向混合學習、一般問題導向學習、機器人程式設計、學習策略、鷹架學習。
而第二篇論文國立陽明交通大學 電子研究所 汪大暉所指導 江宏禮的 嵌入式磁性隨機存取記憶體設計空間分析及其於極低溫金氧半電路之最佳化設計 (2021),提出因為有 磁性隨機存取記憶體、磁性穿隧接面、嵌入式記憶體、極低溫操作、先進金氧半技術、功率-效能-面積分析的重點而找出了 window frame的解答。
最後網站Window Screws - your choice fasteners & tools co., ltd.則補充:Window screws are used to fix PVC frame, door frame. Your Choice offer a broad range of fastening elements, such as self drilling screw and self tapping screw ...
Data Analysis with Python and Pyspark
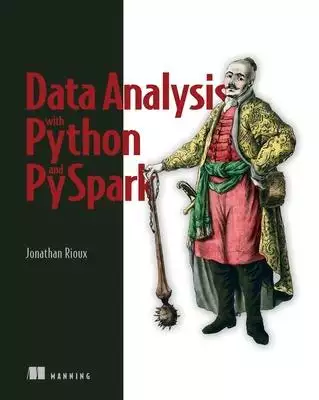
為了解決window frame 的問題,作者Rioux, Jonathan 這樣論述:
Think big about your data! PySpark brings the powerful Spark big data processing engine to the Python ecosystem, letting you seamlessly scale up your data tasks and create lightning-fast pipelines.In Data Analysis with Python and PySpark you will learn how to: Manage your data as it scales acros
s multiple machines Scale up your data programs with full confidence Read and write data to and from a variety of sources and formats Deal with messy data with PySpark’s data manipulation functionality Discover new data sets and perform exploratory data analysis Build automated data pipelines that t
ransform, summarize, and get insights from data Troubleshoot common PySpark errors Creating reliable long-running jobs Data Analysis with Python and PySpark is your guide to delivering successful Python-driven data projects. Packed with relevant examples and essential techniques, this practical book
teaches you to build pipelines for reporting, machine learning, and other data-centric tasks. Quick exercises in every chapter help you practice what you’ve learned, and rapidly start implementing PySpark into your data systems. No previous knowledge of Spark is required. Purchase of the print boo
k includes a free eBook in PDF, Kindle, and ePub formats from Manning Publications. About the technology The Spark data processing engine is an amazing analytics factory: raw data comes in, insight comes out. PySpark wraps Spark’s core engine with a Python-based API. It helps simplify Spark’s steep
learning curve and makes this powerful tool available to anyone working in the Python data ecosystem. About the bookData Analysis with Python and PySpark helps you solve the daily challenges of data science with PySpark. You’ll learn how to scale your processing capabilities across multiple machin
es while ingesting data from any source--whether that’s Hadoop clusters, cloud data storage, or local data files. Once you’ve covered the fundamentals, you’ll explore the full versatility of PySpark by building machine learning pipelines, and blending Python, pandas, and PySpark code. What’s inside
Organizing your PySpark code Managing your data, no matter the size Scale up your data programs with full confidence Troubleshooting common data pipeline problems Creating reliable long-running jobs About the reader Written for data scientists and data engineers comfortable with Python. About th
e author As a ML director for a data-driven software company, Jonathan Rioux uses PySpark daily. He teaches the software to data scientists, engineers, and data-savvy business analysts. Table of Contents 1 Introduction PART 1 GET ACQUAINTED: FIRST STEPS IN PYSPARK 2 Your first data program in PySp
ark 3 Submitting and scaling your first PySpark program 4 Analyzing tabular data with pyspark.sql 5 Data frame gymnastics: Joining and grouping PART 2 GET PROFICIENT: TRANSLATE YOUR IDEAS INTO CODE 6 Multidimensional data frames: Using PySpark with JSON data 7 Bilingual PySpark: Blending Python and
SQL code 8 Extending PySpark with Python: RDD and UDFs 9 Big data is just a lot of small data: Using pandas UDFs 10 Your data under a different lens: Window functions 11 Faster PySpark: Understanding Spark’s query planning PART 3 GET CONFIDENT: USING MACHINE LEARNING WITH PYSPARK 12 Setting the stag
e: Preparing features for machine learning 13 Robust machine learning with ML Pipelines 14 Building custom ML transformers and estimators
window frame進入發燒排行的影片
Don't forget to turn on the bell icon for future uploads 🔔✔️
西洋音樂愛好者✨這裡不會有冗長的介紹文卻是個讓你挖歌的好地方😎
追蹤Gina music社群挖掘更多音樂🌹
facebook👉 https://www.facebook.com/Ginamusicland
instagram👉https://www.instagram.com/ginamusic_yujia/
spotify 歌單👉https://open.spotify.com/playlist/2EfPjFfdqN8NzUwj1XNoZC
kkbox👉https://www.kkbox.com/tw/tc/profile/GqICYlKUZnCZyC0RO7
🌺贊助GINA讓頻道走得更長久•̀.̫•́✧👉https://p.opay.tw/WSwM8
Donate and support my channel (PayPal) 👉https://paypal.me/ginamusic?locale.x=zh_TW
🌸本影片與 Johny Grimes 合作宣傳🌸
想讓更多人認識你的聲音嗎?歡迎投稿😎
Submit your music 👉[email protected]
For business inquiries about copyright issues, photos and song submissions,
please contact👉 https://www.facebook.com/Ginamusicland
____________________________________________________
Social Media:
▶ Download / Stream link : https://www.youtube.com/watch?v=BPUmtcw6caY
👑Johny Grimes
https://soundcloud.com/johny-grimes
https://www.instagram.com/johny_grimes_music/
https://open.spotify.com/artist/32qFjfrKqM9rLXhTbtqblU
https://www.youtube.com/channel/UC0c3dLG6wHmuLx65WYfAPVg
____________________________________________________
Lyrics:
Wait for me
I'm not far behind
I know that i can change
Remembering
All of those nights
Beneath the window frame
Don't know why
We keep going round and round
in the same place
Can't decide
To live my life on the ground or in outer space
Memories
Keep me waiting for you
Leave with me
To the stars above I think I'm ready for you
#JohnyGrimes #Memories #Lyrics #輕電音
範例引導與問題導向混合學習策略對國小學生機器人程式學習成效的影響
為了解決window frame 的問題,作者楊秀全 這樣論述:
問題導向的學習策略已被廣泛地運用在機器人程式設計等跨領域課程,但在此策略中加入範例的引導是否能更具學習成效,是常被關切的課題。本研究以準實驗法,探究「範例引導混合問題導向學習」以及「一般問題導向學習」兩種不同但常用之教學策略的成效,針對95名國民小學五年級學生,進行各14節的機器人程式設計課程。課程前後以自編關鍵能力量表評量學生的自主學習、合作學習、問題解決、批判思考及創造創新等能力。在評量實施後以國際運算思維測驗(Bebras test)評量學生運算思維,和以認知負荷量表評量學生在兩種學習策略下,學習機器人程式設計的認知負荷。研究結果顯示混合範例引導與問題導向學習策略,因為有適切的鷹架範例
導入,可以:提升國小學生的自主學習、合作學習、問題解決以及批判思考等能力,並在學習機器人程式設計上有較好的學習成就,同時提升學生在機器人程式設計的運算思維、降低學生的知負荷。
Excel VBA最強入門邁向辦公室自動化之路王者歸來下冊(全彩印刷)
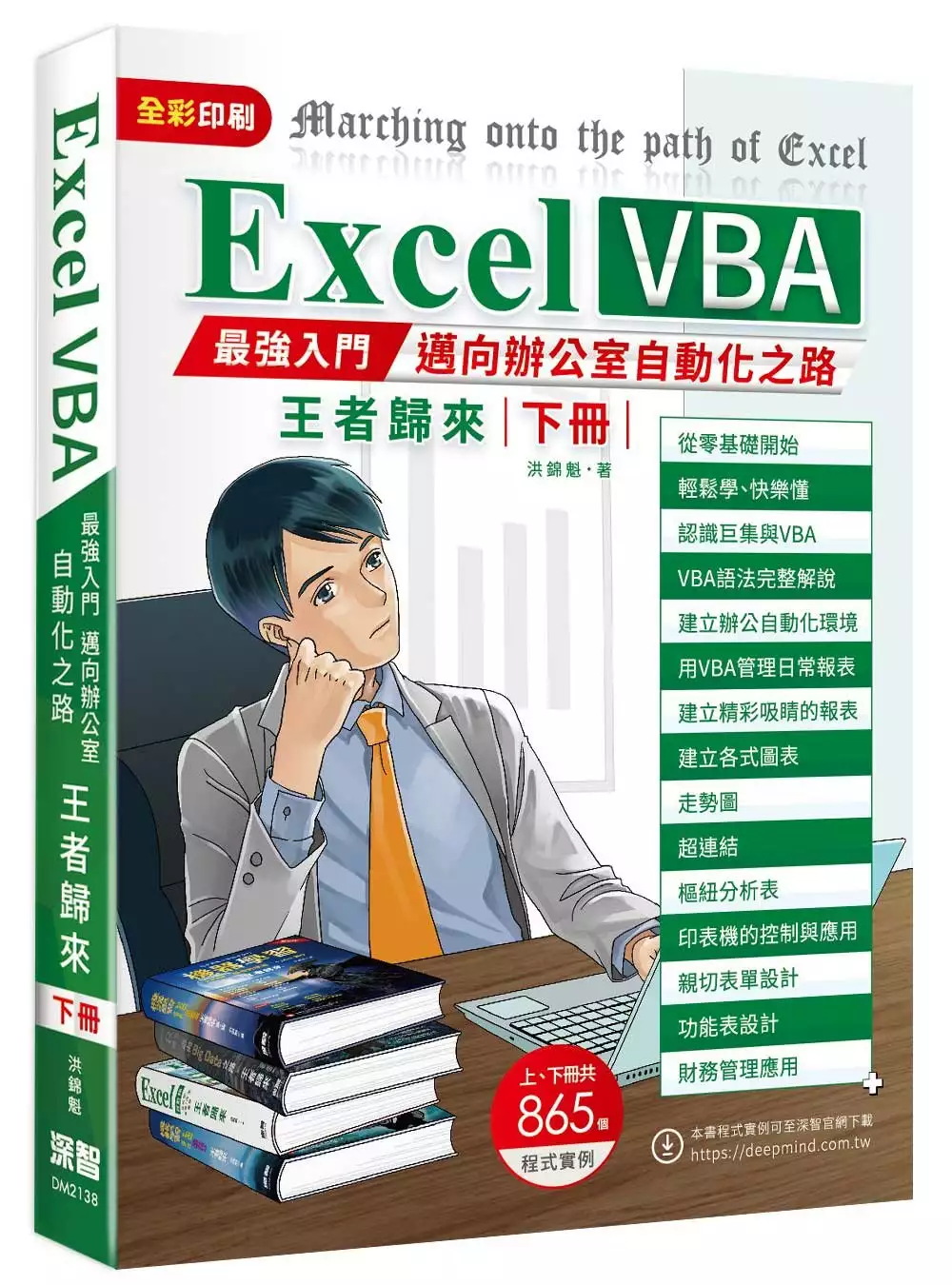
為了解決window frame 的問題,作者洪錦魁 這樣論述:
本書特色 ★★★★★Excel VBA帶領辦公室自動化的最佳著作★★★★★ ☆☆☆☆☆【38個主題】、【865個程式實例】☆☆☆☆☆ 常聽人說Excel VBA不好學,原因是目前沒有一本Excel VBA的中文書是從零開始,逐步與完整解說程式語法、物件與工作表應用的書籍,這也是筆者撰寫這本書的動力來源。 這是國內中文書第一本從零開始帶領讀者完整學習Excel VBA的書籍,【上、下冊】共有38個章節,其中【上冊有18章】著重在認識Excel VBA完整語法與工作表基本操作,【下冊有20章】重點是完整辦公室自動化的應用。除了完整講解VBA語法,每個語法皆有多個程式實例輔
助解說,可以增進讀者學習效率,讀者可以使用VBA學會下列的應用。 ☆【建立與美化工作表】 ☆【徹底了解儲存格】、【工作表】與【活頁簿】 ☆【資料驗證】 ☆【數據統計】、【排序】與【篩選】 ☆【樞紐分析表】 ☆【走勢圖分析】 ☆【視覺化圖表】 ☆【決策分析】 ☆【靜態】與【動態表單設計】 ☆【印表機控制】 ☆【財務管理】 有了上述知識,讀者可以輕鬆將上述觀念應用在【人力】、【財會】、【業務】、【管理】、【分析】等自動化工作。
嵌入式磁性隨機存取記憶體設計空間分析及其於極低溫金氧半電路之最佳化設計
為了解決window frame 的問題,作者江宏禮 這樣論述:
Chinese Abstract iEnglish Abstract iiAcknowledgement iiiContents ivTable Captions vFigure Captions viList of Symbols viiChapter 1 Introduction 11.1 Background 11.2 Description of the Problem 21.3 Organization of this Dissertation
3Chapter 2 Design Space Analysis for Cross-Point 1S1MTJ MRAM: Selector-MTJ Co-Optimization 92.1 Preface 92.2 STT MRAM Used in 1T1MTJ Architecture 102.2.1 Characteristic of MTJ in STT MRAM 102.2.2 Criterion for Read and Write 102.3 Difficulties of 1S1MTJ by Using ST
T MRAM in Current 1T1MTJ MRAM Array 122.3.1 1S1MTJ with Exponential-Type Selector 122.3.2 1S1MTJ with Threshold-Type Selector 142.4 MTJ Optimization for Existing Selectors 152.4.1 Optimization for Exponential-Type Selector 152.4.2 Optimization for Threshold-Type Selector
172.4.3 Endurance Limitation in 1S1MTJ Array 172.5 Design Space Analysis for 1S1MTJ Array 182.5.1 Methodology for Array Level Analysis 182.5.2 Exponential-Type Selector 192.5.3 Threshold-Type Selector 202.6 Summary 21Chapter 3Cryogenic CMOS as a Power-Performance
-Reliability Booster for Advanced FinFETs 423.1 Preface 423.2 FinFET Characterization at Cryogenic Conditions 433.2.1 Long-Channel Mobility Enhancement 433.2.2 Short-Channel Device Characteristics 433.2.3 Reduction of Line Resistance BEOL 453.2.4 Reliability Improveme
nt 453.3 System-Level Analysis 463.3.1 Ring Oscillator Measurement 463.3.2 Power-Performance Analysis 473.3.3 Benefits and Optimization in SRAM 483.4 VTH Design and Adjustment for Cryogenic CMOS 483.4.1 Threshold Voltage Design 483.4.2 Threshold Voltage Adjustme
nt 493.5 Summary 49Chapter 4Cryogenic MRAM as a Density Booster for Embedded NVM in Advanced Technology 714.1 Preface 714.2 MRAM Characterization at Cryogenic Conditions 724.2.1 Steady-State DC Characteristics 724.2.2 Transient AC Characteristics 724.2.3 Thermal S
tability and MTJ Optimization 734.3 Write Analysis and Cell Design for Cryogenic MRAM 744.3.1 Design for Write Voltage/ Current/ Time 744.3.2 Access Transistor Characteristics 754.3.3 Cell Design for Cryogenic MRAM 754.4 Read Margin Analysis 764.3.1 Read Current Distr
ibution and Tailing Bits 764.3.2 Read Margin Enhancement 764.5 Summary 77Chapter 5 Conclusions 94References 96Vita 115Publication List 116
想知道window frame更多一定要看下面主題
window frame的網路口碑排行榜
-
#1.Window Frame - The Chromium Projects
Window Frame. The frame is the area behind the tabs - this is the 'convenient grouping' area for our tabs. We wish to avoid including things within the ... 於 www.chromium.org -
#2.517 520 results for window frame in all - Adobe Stock
Cozy interior of child room with mint armchair, brown mock up poster frame, toys. black metallic window isolated on white background. 於 stock.adobe.com -
#3.window frame 英文中文翻譯- 英漢汽車辭典 - 潤滑油
英文: window frame. 中文: 玻璃框. 常見於汽車系統 > 701 車門及玻璃 > 70155 前擋風玻璃wind shield. 說明: image from http://www.infovisual.info. 於 www.agosto.com.tw -
#4.Window Screws - your choice fasteners & tools co., ltd.
Window screws are used to fix PVC frame, door frame. Your Choice offer a broad range of fastening elements, such as self drilling screw and self tapping screw ... 於 www.ycs.com.tw -
#5.Material for window frames | DECEUNINCK
Window frames made of timber, PVC or aluminium – at Deceuninck you'll find an overview with some practical tips for the window frame to suit you. 於 www.deceuninck.de -
#6.Open Window Frame Images, Stock Photos & Vectors
Find open window frame stock images in HD and millions of other royalty-free stock photos, illustrations and vectors in the Shutterstock collection. 於 www.shutterstock.com -
#7.Window Frame & Parts - Do it Best - DoitBest
Window Frame & Parts ... Sign up today for exclusive offers and expert advice! ... Do it Best has been proudly serving the do-it-yourselfer since 1945. 於 www.doitbest.com -
#8.Glitzhome® Wooden Window Frame - Michaels
Buy the Glitzhome® Wooden Window Frame at Michaels.com. The handcrafted wood frame gives an authentic look and stylish appeal. 於 www.michaels.com -
#9.700+ Free Window Frame & Window Images - Pixabay
Find images of Window Frame. ✓ Free for commercial use ✓ No attribution required ✓ High quality images. 於 pixabay.com -
#10.window frame 中文 - 查查綫上辭典
window frame 中文::窗框;窗口框架…,點擊查查權威綫上辭典詳細解釋window frame的中文翻譯,window frame的發音,音標,用法和例句等。 於 tw.ichacha.net -
#11.24 Main Parts of a Window (Diagrams)
Frame Parts of a Window · 9. Locks · 8. Rails · 7. Stiles · 6. Muntins (grids) · 5. Glass frames (Grilles) · 4. Window Sashes · 3. The head · 2. The jamb. 於 www.wcmanet.org -
#12.How to Build a Window Frame - DIY - PJ Fitzpatrick
If you're in need of a new frame, it only requires a few materials. Here, our window installation experts explain how to build a window frame. 於 www.pjfitz.com -
#13.iron window frames - Alibaba
Find Home Inspiration from the amazing collections. Alibaba.com features elegantly designed iron window frames ideal for all types of uses. 於 www.alibaba.com -
#14.Screen Frame (Small Box) - Metro Screenworks
Window screen frame kit builds three to five window screens 10, six-foot-long sticks of aluminum window screen frame 25 mitered inside window screen corners 60 ... 於 www.metroscreenworks.com -
#15.Types of Window Frames | 2021 Prices and Buying Guide
There are 5 different types of window frame materials for replacement windows that most of the top window brands will offer. These frame types include ... 於 modernize.com -
#16.Wooden Window Frames for sale | eBay
Buy Wooden Window Frames and get the best deals at the lowest prices on eBay! Great Savings & Free Delivery / Collection on many items. 於 www.ebay.co.uk -
#17.Window Framing - Vertica
Window Framing. The window frame of an analytic function comprises a set of rows relative to the row that is currently being evaluated by the function. 於 www.vertica.com -
#18.Types of Windows: Replacement Window Buying Guide - Lowe's
It's secured in an existing window frame with fasteners that run through the window assembly, making it easier to install. When updating existing windows, you ... 於 www.lowes.com -
#19.Farmhouse Window Frame | Hobby Lobby | 1607159
Get Farmhouse Window Frame online or find other Unfinished Wood products from ... Paint or stain this wonderful window, and decorate it to fit your own ... 於 www.hobbylobby.com -
#20.Window Frame - Prime-Line
For the top notch window and screen repair/replacement parts visit Prime-Line. View our current inventory of window frames here. 於 www.primeline.net -
#21.Glazing & Frame Sealants - Screwfix
Acrylic frame sealant is ideal for internal use to fill gaps and cracks between skirting boards, ceiling, windows or door frames. Read more. Sort by. Top ... 於 www.screwfix.com -
#22.Hollow Metal Borrowed Lite Window Frames - Trudoor
4 sided hollow metal frames with fixed glass, which function as a fixed steel window frame. Includes glazing beads and glass - fire-rated glass options. 於 www.trudoor.com -
#23.Succulent Window Frame How To - Salisbury Greenhouse
Create your own beautiful Succulent Window Frame with Salisbury's DIY guide. Succulent Window Frame. Supplies. DIY Step One. DIY Step Two. DIY Step Three. 於 salisburygreenhouse.com -
#24.Window frame definition and meaning - Collins Dictionary
A window frame is a frame around the edges of a window, which glass is fixed into. COBUILD Advanced English Dictionary. Copyright © HarperCollins Publishers. 於 www.collinsdictionary.com -
#25.Robmeg NG4 F7 Steel Window Frame (1511 x 359 x 85mm)
Robmeg NG4 F7 Steel Window Frame (1511 x 359 x 85mm). Builders Warehouse Strubensvalley Change store. R550. Each. (0). Write a review. Regional. 於 www.builders.co.za -
#26.Window Frames - Win32 apps | Microsoft Docs
Most programs should use standard window frames. Immersive applications can have a full screen mode that hides the window frame. 於 docs.microsoft.com -
#27.Guide to Window Frames and Styles - Finance of America ...
A window frame provides support, holding the main parts of your window in place. Quality frames help keep your home insulated and secure the ... 於 www.foahomeimprovement.com -
#28.How Much Does Window Frame Repair Cost in 2021?
Window frame replacement cost; What is the cost to remove windows? Benefits of repairing a window frame; FAQs. If your window frames have seen better days, ... 於 www.checkatrade.com -
#29.Find Out What a Window Frame Is And Why It's Important - DIY ...
A window frame is the window's structural support. Window frames are divided into a head side (the top), sill side (bottom) and jambs (sides). 於 www.diynetwork.com -
#30.12.21.3 Window Function Frame Specification - MySQL ...
A frame is a subset of the current partition and the frame clause specifies how to define the subset. Frames are determined with respect to the current row, ... 於 dev.mysql.com -
#31.You've Been Framed - The Importance of a Replacement ...
Vinyl replacement window frames are designed to support that additional weight. Connection—The window frame is the connection between the glass and the home ... 於 www.sunrisewindows.com -
#32.window frame 中文意思是什麼
window frame 中文意思是什麼. window frame 解釋. 窗框,窗架。 window : n 1 窗;窗口,窗戶;窗扉,窗框;窗玻璃;(商店的)櫥窗;【計算機】窗口〈電腦視頻顯示器 ... 於 dict.site -
#33.Window Frame Open Icon | Font Awesome
I'm afraid I can't window-frame-open that, Dave. Retry? TODOs. Collect underpants Set up Font Awesome 5 Use window-frame-open in a new project Profit! Home. 於 fontawesome.com -
#34.DIY Window Frame - Frills and Drills
DIY tutorial for framing out a window. From builder grade to custom design. 於 www.frillsanddrills.com -
#35.Window.frames - Web API 接口参考| MDN
在window.frames类数组中的每一项都代表了窗口对应给定对象的<frame>或<iframe>的内容,而不是(i)frame DOM元素(即window.frames[ 0 ]与document. 於 developer.mozilla.org -
#36.Windows - The Home Depot
Also called retrofit or insert windows, Replacement Windows can be installed without cutting out the existing window siding and frame. Some New Construction ... 於 www.homedepot.com -
#37.14.4. Fixing a window frame
INTRODUCTION: This section teaches students the names of the parts of a window frame, calculation of an opening for a window frame and how window frames are ... 於 www.nzdl.org -
#38.Window frame - Energy Education
The window frame is the portion of the window that holds the glazing and sits between the glazing and the wall of the building when ... 於 energyeducation.ca -
#39.window frame - Yahoo奇摩字典搜尋結果
窗框;窗架. Dr.eye 譯典通片語. window frame. 美式. ph. 窗框;窗架. Dr.eye 譯典通. window frame. ph. 窗框. 牛津中文字典. window frame. ph. 窗框窗口框架 ... 於 tw.dictionary.yahoo.com -
#40.9 Common Window Frame Issues
1. Difficulty Opening Windows · 2. Excessive Window Condensation · 3. Drafty Windows · 4. Rotting Window Frames · 5. Broken Window Panes · 6. Leaking ... 於 homespirewindows.com -
#41.Window Frame Replacement, Repair & Installation - Magic ...
If the frame of your window is broken, we are here to help you replace it quickly and efficiently. Regardless of the design or material used, ... 於 magicglass.com.au -
#42.door or window frame - 英中– Linguee词典
大量翻译例句关于"door or window frame" – 英中词典以及8百万条中文译文例句搜索。 於 cn.linguee.com -
#43.Window Frame - Walmart.com
Shop for Window Frame at Walmart.com. Save money. Live better. ... 2 Pack Rustic White Wood Window Frame for Farmhouse Home Wall Decor, 11 x 15 in. 於 www.walmart.com -
#44.Universal Window Frame Kit - Accessories - Building Kits
2" x 3"; 15GA. For 2" x 3" and 2" x 4" frame structures. Adjustable for 4', 5' or 6' on center truss spacing and 48"H or 60"H window openings. 於 www.versatube.com -
#45.Window frame Materials & Design - GreenSpec
Heat lost through a window frame in its life > than the energy used to manufacturer it (embodied energy) Design with materials with low thermal ... 於 www.greenspec.co.uk -
#46.Full-Frame Window Replacement Installation
Full-frame replacement windows mirror new construction installations. The entire window frame and sash are removed, resulting superior weather tightness and ... 於 www.andersenwindows.com -
#47.Adler 2 Window Frame - Country Road
Looking for a Black Adler 2 Window Frame? Country Road has the latest styles in Frames. Free delivery over $100 & 60-day returns. Shop now. 於 www.countryroad.com.au -
#48.Ultimate Casement Narrow Frame | Marvin
The Ultimate Casement Narrow Frame window is a sleek contemporary style that allows for easy installation without disturbing the existing frame or wall. 於 www.marvin.com -
#49.window frame - Translation into Chinese - examples English
Translations in context of "window frame" in English-Chinese from Reverso Context: At a video of the latest site > Robert Akiyama of the window frame. 於 context.reverso.net -
#50.Window Frame Installer Skilled Trade Test Mock Practical Test ...
four sides of window frames to ensure absence of water seepage; ... Installing a planar steel window frame (W2) according to Working. 於 cic.hk -
#51.CRL Window Frame and Corners - C.R. Laurence
C. R. Laurence is the world leader, wholesale distributor to the Glazing, Industrial, Construction, Architectural, Hardware and Automotive Industries, ... 於 www.crlaurence.com -
#52.24 Window Frames ideas
Mar 6, 2013 - Different window frames designed to fit everyone's lifestyle. See more ideas about window frames, frame design, design. 於 www.pinterest.ph -
#53.Window Frame Repair | Mr. Handyman
Expert Window Frame Repair. Are Your Window Frames Looking Worse for the Wear? Due to their nature, windows are exposed to the elements. 於 www.mrhandyman.com -
#54.WINDOW FRAME (noun) definition and synonyms - Macmillan ...
Definition of WINDOW FRAME (noun): the frame around the edge of a window. 於 www.macmillandictionary.com -
#55.塑鋼
塑鋼. vinyl window and door frame material. 概觀. 塑鋼邊框是一種節能材料,由於它的導熱性能較 ... 於 www.valuewindowsdoors.com -
#56.Your Guide to the 4 Most Common Window Frame Materials
Your selection of window frame material will impact the visual appeal, energy efficiency, and maintenance needs of your windows throughout their lifetime. 於 chicagowindowguy.com -
#57.Frame and Dividers - DesignBuilder
A frame surrounds the glazing in a window (schematics below). It is assumed that all frame characteristics, such as width, conductance and solar absorptance, ... 於 designbuilder.co.uk -
#58.How to paint a wooden window frame | Ideas & Advice - B&Q
A tired looking window stands out even more next to a freshly painted wall. Our 'Do It In A Day' projects is perfect for tidying up interior sills and frames to ... 於 www.diy.com -
#59.Origin Global's High Quality Bespoke Fixed frame Windows
Introducing theOrigin Fixed Window · Our Fixed Frame Windows are a perfect option for bringing more light into your home or adding a picturesque frame to an ... 於 origin-global.com -
#60.Window-frame - The Free Dictionary
Define window-frame. window-frame synonyms, window-frame pronunciation, window-frame translation, English dictionary definition of window-frame. n. 1. a. 於 www.thefreedictionary.com -
#61.Window Frames Steel - Cashbuild
Window Frame Steel C1h F7 Left Hand. R399.95. 於 www.cashbuild.co.za -
#62.Frame Materials - Milgard Windows
Browse window and door frame materials from Milgard. See which material works best for your home. Explore Milgard's window or door frame materials today. 於 www.milgard.com -
#63.Energy efficient home window frames | Sustainability Victoria
The most energy efficient window frames are made from materials that won't transfer heat and cold, such as timber, uPVC or combination ... 於 www.sustainability.vic.gov.au -
#64.Plastic Window Frame System "Eco", 17 mm profile - VKF ...
Plastic Window Frame System "Eco", 17 mm profile | View our full range and order securely online | Fast delivery | VKF Renzel – The POS experts since 1985. 於 www.vkf-renzel.com -
#65.Window Frame - Amazon.com
Price and other details may vary based on product size and color. ... Farmhouse Window Pane Wall ... 於 www.amazon.com -
#66.Distressed Window Frame - Target
Shop for distressed window frame online at Target. Choose from contactless Same Day Delivery, Drive Up and more. 於 www.target.com -
#67.How to Specify a Window Frame | Architect Magazine
But what differentiates one window assembly from the next? Materials Standard materials for commercial window frames are vinyl, aluminum, fiberglass, wood, and ... 於 www.architectmagazine.com -
#68.Window frame screw - fischer fixings
The fischer window frame screw is a special screw (with a length of up to 302 mm) for the cost-effective installation of window frames without plugs. 於 www.fischer.co.uk -
#69.30,000+ Window Frame Pictures | Download Free Images on ...
Download the perfect window frame pictures. Find over 100+ of the best free window frame images. Free for commercial use ✓ No attribution required ... 於 unsplash.com -
#70.What Is a Window Frame? - Thompson Creek
Window frames surround and support the entire window system with an operational or stationary framework that includes the head, ... 於 www.thompsoncreek.com -
#71.Window Types and Technologies | Department of Energy
To reduce heat flow, metal frames should have a thermal break -- an insulating plastic strip placed between the inside and outside of the frame and sash. 於 www.energy.gov -
#72.Window frame with Keystone and Windowsill 窗框頂石與窗台 ...
HomeWindowWindow frame with Keystone and Windowsill 窗框頂石與窗台|FutureView360. feel free to call us +886.973.737638 [email protected] ... 於 www.futureview360.com -
#73.Window Functions - Snowflake Documentation
What is a Window Frame? Types of Window Frames. What is a Rank-related function? Window Syntax and Usage. 於 docs.snowflake.com -
#74.What are the Different Parts of a Window Called? - JELD-WEN ...
Most windows have two main components, the frame and the sash. The frame is the outermost area, or casing of the window, and inside it are ... 於 inspiration.jeld-wen.ca -
#75.Wooden Window Frame For Sale | Buco
To see inventory and prices choose a store. Shop Wood Window Frames Online. For the widest range of wooden window frames at great prices, ... 於 www.buco.co.za -
#76.6 Common Window Materials for Your Home - Banner ...
Knowing the different types of window frame materials will guide homeowners in selecting the right one for their home. 於 bannerconstruction.com -
#77.Window Frame | Etsy
Check out our window frame selection for the very best in unique or custom, handmade pieces from our wall decor shops. 於 www.etsy.com -
#78.In The Window Frame-歌詞-Nicklas Sahl|MyMusic 懂你想聽的
In The Window Frame-歌詞-無歌詞-MyMusic懂你想聽的,打開APP立即開始聽歌. 於 www.mymusic.net.tw -
#79.What Is The Best Window Frame Material?
Modern window frames come in various material options, including vinyl, aluminum, composite, traditional wood, wood-clad, etc. The critical question to ask ... 於 superiorwindowcompany.com -
#80.Window frames Property - W3Schools
Window frames Property. ❮ Window Object. Example. Change the location of the first <iframe> element (index 0) in the current window:. 於 www.w3schools.com -
#81.Window Frame Pictures, Images and Stock Photos - iStock
Search from 70224 Window Frame stock photos, pictures and royalty-free images from iStock. Find high-quality stock photos that you won't find anywhere else. 於 www.istockphoto.com -
#82.Window Frame Images | Free Vectors, Stock Photos & PSD
Find & Download Free Graphic Resources for Window Frame. 13000+ Vectors, Stock Photos & PSD files. ✓ Free for commercial use ✓ High Quality Images. 於 www.freepik.com -
#83.How to Make Frames (Main Windows) - Oracle Help Center
A frame, implemented as an instance of the JFrame class, is a window that has decorations such as a border, a title, ... 於 docs.oracle.com -
#84.CECO Window Frame Kit, 24x30 In - 3PEU8 - Grainger
Technical Specs · Item Door Window Frame Kit · Material 20-Gauge Galvannealed Steel · Finish Galvannealed · Opening Height 32 in · Opening Width 26 in · Window Height ... 於 www.grainger.com -
#85.413 幅Window Frame插畫檔、美工圖案、卡通及圖標 - Getty ...
尋找Getty Images 帶來的完美Window Frame插畫檔。從413 項非凡卓絕的優質Window Frame圖片當中任意挑選。 於 www.gettyimages.hk -
#86.Window Frame - Graphisoft
Window Frame This group contains the settings for the frame. The tab Frame can be found in the Window Default Settings menu under the Storefront Settings ... 於 graphisoft.com -
#87.The Parts of a Window | Pella
A window frame is the framework that surrounds and supports the entire window system. It is comprised of the head, jamb and sill. Head The head is the main ... 於 www.pella.com -
#88.What in the World is a Window Frame?
what is a window frame? A window frame is the combination of the head, jambs and sill that forms a precise opening in which a window sash fits. 於 www.simonton.com -
#89.Aluminum Window Frame - IndiaMART
Find here Aluminum Window Frame, Aluminium Window Frame manufacturers, suppliers & exporters in India. Get contact details & address of companies ... 於 dir.indiamart.com -
#90.Old Window Frame Wall Decor | Wayfair
Shop Wayfair for the best old window frame wall decor. Enjoy Free Shipping on most stuff, even big stuff. 於 www.wayfair.com -
#91.Window Frame Single Rebate (70mm x 57mm) - Terry's Timber
Machined from selected redwood are single rebate window frame is ideal to replace old existing frames or make new sash windows. Delivery in Liverpool and ... 於 www.terrystimber.co.uk -
#92.30 Parts of a Window and Window Frame (Diagrams) - Home ...
Second illustrations is a cross section of a 3-pane window labeling all the different parts of the window including the frame. When you're replacing windows, ... 於 www.homestratosphere.com -
#93.Window Frame Kit Abs Accessories Search - Maruti Suzuki
Query : Window-Frame-Kit-Abs. Showing 1 – 20 of 365 results. Sort By. FILTERS. Clear All. CATEGORIES. Exterior. Roof Luggage Carrier. Door Handle. 於 www.marutisuzuki.com -
#94.Residential Aluminium Awning Window | Vantage | AWS
50mm aluminium frame. Perfect for single or double glazing. A huge variety of performance-glass options to maximise energy efficiency. Can be ... 於 www.awsaustralia.com.au -
#95.Window - Wikipedia
Modern windows are usually glazed or covered in some other transparent or translucent material, a sash set in a frame in the ... 於 en.wikipedia.org