Workflow diagram的問題,透過圖書和論文來找解法和答案更準確安心。 我們找到下列懶人包和總整理
Workflow diagram的問題,我們搜遍了碩博士論文和台灣出版的書籍,推薦Caroli, Paulo寫的 Cumulative Flow Diagram: A valuable tool for improving workflow 可以從中找到所需的評價。
另外網站Flowchart Maker & Online Diagram Software也說明:diagrams.net is free online diagram software for making flowcharts, process diagrams, org charts, UML, ER and network diagrams.
長庚大學 醫務管理學系 許績天、趙銘崇所指導 羅佳玲的 巡迴體檢中心導入資訊化作業流程人力探討 (2021),提出Workflow diagram關鍵因素是什麼,來自於巡迴健康檢查、醫療資訊化、個案研究。
而第二篇論文臺北醫學大學 國際醫學研究博士學位學程 康峻宏、黎阮國慶所指導 TRUONG NGUYEN KHANH HUNG的 運用深度學習於膝關節損傷核磁共振影像之人工智慧偵測與診斷模型 (2021),提出因為有 Artificial intelligence、deep learning、machine learning、Knee MRI、ACL、meniscus的重點而找出了 Workflow diagram的解答。
最後網站Guide to Process Diagramming [+Templates] - Venngage則補充:Process diagrams can help ensure a task is performed the same way over time and across a team. They ...
Cumulative Flow Diagram: A valuable tool for improving workflow
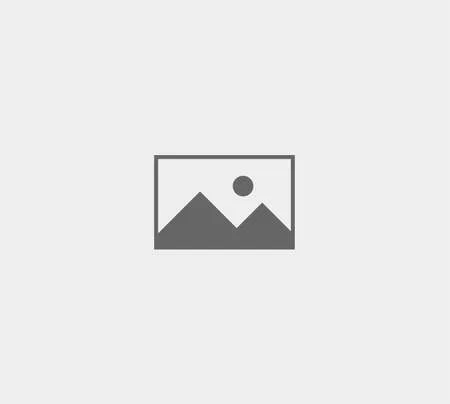
為了解決Workflow diagram 的問題,作者Caroli, Paulo 這樣論述:
巡迴體檢中心導入資訊化作業流程人力探討
為了解決Workflow diagram 的問題,作者羅佳玲 這樣論述:
中文摘要 iAbstract ii表目錄 v圖目錄 vi第一章 緒論 1第一節 研究背景與動機 1第二節 研究目的 6第二章 文獻探討 7第一節 勞工健康檢查 7第二節 醫療資訊化系統 12第三節 以資訊化系統改善作業流程之應用 15第三章 研究方法 19第一節 研究設計 19第二節 研究流程 23第三節 研究對象 23第四章 研究結果 25第一節 個案醫院介紹 25第二節 個案研究步驟 30第三節 資訊化作業規劃 40第四節 效益評估 44第五章 結論與建議 46第一節
結論 46第二節 管理意涵 46第三節 建議 47第四節 研究限制 48參考文獻 50附件 58表目錄表一 受訪者名單 24表二 巡迴體檢中心客戶結構 28表三 巡迴體檢現場各站別作業面問題彙整 34表四 巡迴體檢業務內容分析 35表五 巡迴體檢中心企業合作產業 36表六 巡迴體檢中心護理師及庶務員人力時間計算 39表七 資訊化作業流程彙整 40表八 廠商規劃新增資訊化功能 42表九 巡檢資訊化改善後人力節省 44圖目錄圖一 巡迴體檢中心年度收入與人力編制 26圖二 巡迴體檢中心收入及服務量 26圖三 2020 年及 2021 年巡迴體檢中心收入影響 27
圖四 巡迴體檢作業流程 29圖五 巡迴體檢現場作業流程模擬圖 29圖六 特性要因圖 38圖七 巡檢資訊化系統架構圖 43
運用深度學習於膝關節損傷核磁共振影像之人工智慧偵測與診斷模型
為了解決Workflow diagram 的問題,作者TRUONG NGUYEN KHANH HUNG 這樣論述:
Introduction: Efficient and accurate detection is vital for the diagnosis and treatment of knee injuries. In recent years, there is an increase in interest in deep learning (DL) approaches to detecting knee injuries in magnetic resonance imaging (MRI). Studies have shown that DL models are capable
of reaching the same level as human radiologists when it comes to sensitivity and specificity, while at the same time requiring significantly less training time. Current Artificial Intelligent (AI) - based systems are, however, still limited by many different factors, such as unbalanced classes in t
raining data, or the nature of these systems which makes false positives and false negatives almost an inevitability. There are multiple routes for improving upon the existing DL knee injury detection models. As they continue to become more and more advanced, it is expected that the use of these sys
tems will become more popular in the future.Method: In this study, we create multi models based on machine learning (ML) and DL algorithms to perform classification, recognition, and segmentation tasks on knee MRI. In which the two most important components in the knee joint in this study are the an
terior cruciate ligament (ACL) and meniscus.The first model, based on the DenseNet 121 neural network structure, was used to classify images with or without ACL injury. The dataset includes 799 knee MRI reports from Cho Ray Hospital (Vietnam). These MRI data were obtained from previous work in the h
ospital, containing knee MRI reports from 5 years (January 1st, 2015 – December 31st, 2019)Using the Faster-region convolutional neural network (Faster - RCNN) and several convolutional neural networks (CNN) backbone tests, such as VGG-16, Res-Net50, DenseNet-121, EfficientNet-B0, and EfficientNetV2
- B0 algorithms, the second group of models can recognize the ACL on knee MRI as a function of the typical imaging characteristics. This research collected 256 knee MRI examinations performed at Cho Ray Hospital, Ho Chi Minh City, Vietnam, between January 1, 2018, and December 31, 2020 (including t
raining and testing datasets).The third model focuses on automatic identification and classification of meniscus based on the Yolo-v4 object detection model. At the same time, the lesion location is also shown on images by the GRAD-CAM technique. The total number of subjects used in this study was 7
04 patients, including meniscus lesions and the control group. All MRIs in this study were collected before the surgery, and all had no prior surgical history. The MRI scanner at Cho Ray Hospital is MAGNETOM Skyra 3T (Siemen), and at Hoan My Hospital is 3.0T MRI Scanners SIGNA (GE Healthcare). In ad
dition, we also used a public dataset - MRNet dataset (validation dataset) from Stanford University Medical Center with 120 examinations for external testing.Results: The area under the ROC curve (AUC) for the ACL injury classification system was 80.63% with the axial plane and around 78% with both
the sagittal and coronal planes, respectively. All sensitivity and specificity point estimates of the proposed ACL injury detection system were all over 96%, indicating no significant differences in diagnostic performance between different planes.Our DL model detected meniscus tears with 91.4% accur
acy on the internal testing dataset, 89.2% accuracy on the external validation dataset, and 79.9% accuracy on the MRNet dataset, respectively. The meniscus tears were visualized by auto-enlarging the detection area and Grad-CAM images.Conclusion: This report describes the various approaches in knee
MRI experiments to provide different AI models for the prediction of knee injuries. The CNN model applied to classify injured ACL images had high sensitivity and specificity, showing that using a simple structured 2D-CNN is more effective for small datasets and can assist non-experts in assessing th
e assessment of ACL injuries. The proposed model applied to detect meniscus lesions had high accuracy and specificity, showing that our model can assist non-experts in assessing the assessment of meniscus injuries.
想知道Workflow diagram更多一定要看下面主題
Workflow diagram的網路口碑排行榜
-
#1.How to Create a Workflow Diagram - with examples! | Gmelius
A workflow diagram is a visual representation of all the steps within a business process from start to finish. It shows the chronological flow ... 於 gmelius.com -
#2.Workflow Modeling with UML Diagrams - CEG - Corporate ...
The principle diagram for workflow modeling is the Activity Diagram. While the basic notation looks similar to the traditional flow chart, it does contain ... 於 www.corpedgroup.com -
#3.Flowchart Maker & Online Diagram Software
diagrams.net is free online diagram software for making flowcharts, process diagrams, org charts, UML, ER and network diagrams. 於 app.diagrams.net -
#4.Guide to Process Diagramming [+Templates] - Venngage
Process diagrams can help ensure a task is performed the same way over time and across a team. They ... 於 venngage.com -
#5.How to Diagram a Workflow - Bridging the Gap
7 Steps to Diagram a Workflow · Identify the process. · Name your process. · Identify a clear start point and end point · Identify your purpose for ... 於 www.bridging-the-gap.com -
#6.Business process flow diagram: How to make one in 6 simple ...
A business process flow diagram is the simplest and most primary representation of processes. It serves to initiate a further and more complex ... 於 www.heflo.com -
#7.先別急著畫UI,你聽過Flow Chart嗎
Logic Flow也可以說是操作邏輯流程,常常也會聽到許多不同名詞,例如Task Flow、User Flow、Flow Chart等等,這些其實都是Flow,也就是為了了解流程而 ... 於 medium.com -
#8.Process Flow Diagrams | BPI Consulting - SPC for Excel
A process flow diagram is a detailed picture of a process. It has many uses. It is a great technique for determining where a process should be measured. It also ... 於 www.spcforexcel.com -
#9.Workflow Diagram Examples & Best Practices | Pipefy
Best practice: Workflow diagrams can get quite large and have a tendency to sprawl, depending on the scope of the process and the amount of detail you want to ... 於 www.pipefy.com -
#10.Process Flow Diagram Tool - Visual Paradigm
An Easy Process Flow Diagram maker that features a full library of PFD examples and PFD tools. Easily create your own Process Flow Diagrams with ... 於 www.visual-paradigm.com -
#11.Workflow Diagram Definition - EA Method - Dragon1
A Workflow Diagram is a visualization of work (a series of actions, activities, usage of resources and decisions). It shows how work is done or should be ... 於 www.dragon1.com -
#12.Work-Flow Chart: Example & Definition - Study.com
A workflow chart, or diagram, is a visual representation of the different steps in a business process. Define a workflow diagram through an. 於 study.com -
#13.Shit flow diagrams (SFD) - Sustainable Sanitation Alliance
An excreta flow diagram (or shit flow diagram, SFD) is a tool to readily understand and communicate how excreta 'flow' through a city or town. 於 www.susana.org -
#14.How to draw a Workflow Diagram | MyDraw
Workflow diagrams are a visual representation of a work process. Most commonly used for implementation or documentation purposes, the diagrams help describe ... 於 www.mydraw.com -
#15.Step-by-Step Guide for Creating a Workflow Diagram - Kuali ...
A workflow diagram is a visual representation of a business process. It is most commonly depicted using shapes and symbols in a flowchart ... 於 build.kuali.co -
#16.FLOW DIAGRAM在劍橋英語詞典中的解釋及翻譯
flow diagram 的意思、解釋及翻譯:flow chart。了解更多。 於 dictionary.cambridge.org -
#17.Create and manage workflow diagrams - ArcGIS Enterprise
Workflow diagrams allow you to create a visual representation of a business process that consists of steps and paths. Complete the steps in the sections ... 於 enterprise.arcgis.com -
#18.Process Flow Chart Tutorial - MoreSteam
The Process Flow chart provides a visual representation of the steps in a process. Flow charts are also referred to as Process Mapping or Flow Diagrams. 於 www.moresteam.com -
#19.What is the significance of a flowchart? [UPDATED]
A flowchart is a diagram depicting a process, a system or a computer algorithm. It is a diagrammatic representation of the solution to a given problem but, ... 於 www.ucl.ac.uk -
#20.System workflow diagram. - ResearchGate
Download scientific diagram | System workflow diagram. from publication: Standard Distance Detector Tool for TV users based on Fuzzy Decision Tree | Eye ... 於 www.researchgate.net -
#22.STARD 2015 flow diagram (PDF) - The EQUATOR Network
Page 1. Prototypical STARD diagram to report flow of participants through the study. 於 www.equator-network.org -
#23.Material flow diagram - Circular economy - Eurostat
Material flow diagram. This Sankey diagram shows the flows of materials as they pass through the EU economy and are eventually discharged back into the ... 於 ec.europa.eu -
#24.Wireframing User Flow with Wireflows - Balsamiq
There are several types of flow diagrams that are useful in designing software products — task flows, user flows, and flowcharts. The lines between each of ... 於 balsamiq.com -
#25.Workflow Diagram - Creately
Workflow diagram to visualize the flow of work through an organization. Click to customize this workflow diagram online and add details relevant to your ... 於 creately.com -
#26.What is a workflow diagram? - Quora
A workflow diagram is a visual representation of a business process (or workflow), usually done through a flowchart. It uses standardized symbols to ... 於 www.quora.com -
#27.Workflow Diagram - Definition and How to Create One
A Workflow Diagram offers a bird's eye view of the business and its processes. It is a visual depiction of your standard workflow as it moves between resources. 於 getbreakout.com -
#28.The 7 Best Flowchart Software of 2021 - The Balance Small ...
Flowchart software makes it easy to create workflows, diagrams, and charts in minutes. We reviewed the best flowchart software programs ... 於 www.thebalancesmb.com -
#29.What is a Workflows Process Diagram? | Klipfolio
A workflow diagram provides a visual step-by-step overview of your work sequence from start to finish using symbols and shapes. 於 www.klipfolio.com -
#30.Cumulative Flow Diagram - Workfront
Cumulative flow diagrams monitor workflow, and anticipate bottlenecks to adjust workflow accordingly, and help make processes more predictable. Learn more. 於 www.workfront.com -
#31.Flow Charts - Reference For Business
Flow charts are graphical representations of a process that illustrates the sequence of steps needed to reach the process's outcome. A flow chart may ... 於 www.referenceforbusiness.com -
#32.flow diagram; process flow chart - 流程圖 - 國家教育研究院雙語 ...
出處/學術領域, 中文詞彙, 英文詞彙. 學術名詞 海事, 流程圖, flow chart; flow diagram; process flow chart. 學術名詞 畜牧學, 流程圖, process chart. 學術名詞 於 terms.naer.edu.tw -
#33.Data Flow Diagram, workflow diagram, process flow diagram
Data Flow Diagrams are intended for graphical representation of data flows in the information system and for analysis of data processing during the structural ... 於 www.conceptdraw.com -
#34.The CONSORT Flow Diagram
Flow diagram of the progress through the phases of a parallel randomised trial of two groups (that is, enrolment, intervention allocation, follow-up, ... 於 www.consort-statement.org -
#35.Flowchart - Wikipedia
A flowchart is a type of diagram that represents a workflow or process. A flowchart can also be defined as a diagrammatic representation of an algorithm, ... 於 en.wikipedia.org -
#36.Cumulative Flow Diagram for Best Process Stability - Kanbanize
The cumulative flow diagram (also known as CFD) is one of the most advanced Kanban and Agile analytics charts. It provides a concise visualization of the three ... 於 kanbanize.com -
#37.What Are Workflow Diagrams? | IBM
A workflow diagram is a visual layout of a process, project or job in the form of a flow chart. It's a highly effective way to impart the steps ... 於 www.ibm.com -
#38.PRISMA Flow Diagram
PRISMA Flow Diagram. The flow diagram depicts the flow of information through the different phases of a systematic review. It maps out the number of records ... 於 prisma-statement.org -
#39.Workflow Diagram - How to Create Workflow Chart - SmartDraw
A workflow diagram depicts a series of actions that define a job or how work should be done. A workflow diagram visualizes how tasks will flow between ... 於 www.smartdraw.com -
#40.What is a Data Flow Diagram (DFD)? - SearchDataManagement
Learn about data flow diagrams, including how they are used and how to create a DFD. Discover the difference between logical and physical DFDs. 於 searchdatamanagement.techtarget.com -
#41.Flow Chart - Vecta.io
General flow chart symbols. 於 vecta.io -
#43.How to Read the Cumulative Flow Diagram [Infographic] | Nave
In this infographic, we'll be explaining how to read the cumulative flow diagram to track the stability of your process by measuring the main flow metrics. 於 getnave.com -
#44.What is a Workflow Diagram: Definition and 3+ Examples
A workflow diagram is a visual representation of a business process (or workflow), usually done through a flowchart. It uses standardized symbols to describe ... 於 tallyfy.com -
#45.Create a basic flowchart in Visio - Microsoft Support
Flowcharts are diagrams that show the steps in a process. Basic flowcharts are easy to create and, because the shapes are simple and visual, they are easy ... 於 support.microsoft.com -
#46.Flow Chart Diagram | Enterprise Architect User Guide - Sparx ...
The Flow Chart Diagram is a strategic diagram that allows the steps in a process, workflow or algorithm to be ordered in a sequence and displayed graphically. 於 sparxsystems.com -
#47.Workflow Template | MURAL
A workflow diagram visualizes the actions and steps needed to complete a project or process. At their simplest level, workflow diagrams are flowcharts ... 於 www.mural.co -
#48.Proposal Workflow Chart | Research Administration - UMass ...
At right, download a diagram that depicts the proposal workflow steps through OPAS. Last modified: December 1, 2021. Proposal Related. 於 www.umass.edu -
#49.What is a Workflow? A Simple Guide to Getting Started
Workflows are the way people get work done, and can be illustrated as series of steps that need to be completed sequentially in a diagram or ... 於 www.process.st -
#50.GTD Workflow Processing and Organizing - Getting Things ...
GTD Workflow Processing and Organizing. 11. +. I. LIFE'S RANDOM INPUTS. \ ]. "STUFF". Purpose. Vision. WHAT IS IT? WHAT IS IT? DEFINING WORK. 於 gettingthingsdone.com -
#51.Design Workflow Diagrams and Flowcharts - Grapholite
Workflow diagram usually denotes movement of documents, resources, and data throughout the entire work process for a specific product or service. 於 grapholite.com -
#52.Using the visualization graph - GitHub Docs
Every workflow run generates a real-time graph that illustrates the run progress. You can use this graph to monitor and debug workflows. 於 docs.github.com -
#53.Business Process Workflow Diagram Powerpoint Slide ...
Looking for Business Process Workflow Diagram Powerpoint Slide Influencers PowerPoint templates? Find predesigned PPT templates, presentation slides ... 於 www.slideteam.net -
#54.Find Your Flow: Process Flow Diagrams for Beginners - Six ...
You can use Process flow diagrams in just about any industry to organize process steps in sequential order. The process flow diagram is ... 於 www.6sigma.us -
#55.Cumulative Flow Diagram | Kanban Tool
Cumulative Flow Diagram is the most insightful analysis associated with Kanban. It helps you see the workflow at one glance and identify any problems ... 於 kanbantool.com -
#56.Study flow diagrams in Cochrane systematic review updates
Cochrane systematic reviews are conducted and reported according to rigorous standards. A study flow diagram must be included in a new ... 於 pubmed.ncbi.nlm.nih.gov -
#57.A Beginner's Guide to Data Flow Diagrams - HubSpot Blog
Evaluate your organization's process with data flow diagrams and your company will have a firm grasp on how to perfect various processes and ... 於 blog.hubspot.com -
#58.Flow Chart Vector Art, Icons, and Graphics for Free Download
Browse 9704 incredible Flow Chart vectors, icons, clipart graphics, and backgrounds for royalty-free download from the creative contributors at Vecteezy! 於 www.vecteezy.com -
#59.9 Functional Flow Diagram ideas - Pinterest
Nov 9, 2018 - Explore Alfred Pizzurro's board "Functional Flow Diagram" on Pinterest. See more ideas about flow chart, process flow, process flow chart. 於 www.pinterest.com -
#60.Flowchart | IHI - Institute for Healthcare Improvement
A high-level flowchart, showing six to 12 steps, gives a panoramic view of a process. These flowcharts show clearly the major blocks of activity, ... 於 www.ihi.org -
#61.Fix hidden tasks in workflow diagram view - Collibra - Developer
In this tutorial you will learn how to fix hidden tasks in the Eclipse workflow diagram view. This sometimes happens when you move a task to a another ... 於 developer.collibra.com -
#62.Free Online Flowchart Maker for Professional ... - Visme
Visme offers easy flow diagramming in minutes. ... The flowchart maker will let you create a professional flow diagram or org chart just how you need it. 於 www.visme.co -
#63.Revisiting your daily routine workflow diagram if you want to ...
This is a habit I developed over the last 15 years working on process automation/workflow engine projects. This diagram is a great prototype of ... 於 blog.bernd-ruecker.com -
#64.A Workflow Diagram: What It Is and How to Create It | Blog
A workflow diagram is a graphic overview of a business process, most commonly done through a flowchart. It shows the steps needed to ... 於 whatagraph.com -
#66.Flowchart Examples & Templates Teams Love | Miro
A flowchart is a visual representation of a process using shapes & arrows to indicate ... Timeline Workflow Thumbnail ... Data Flow Diagram Maker Template. 於 miro.com -
#67.Workflow Diagram - an overview | ScienceDirect Topics
Formal process flow diagrams are often called process maps, activity diagrams, or workflow diagrams. Historically, process analysts have used a wide variety of ... 於 www.sciencedirect.com -
#68.What is A Workflow Diagram with Examples | EdrawMax Online
“A Workflow Diagram is a visual representation of the said workflow, or business processes and activities such as movement or transfer of data, documents, ... 於 www.edrawmax.com -
#69.Flowchart Template | Beautiful.ai
A flowchart is a diagram that shows the sequence of steps and decisions needed to perform a process. Each step in the sequence is noted within a diagram ... 於 www.beautiful.ai -
#70.Workflow Diagrams and Charts - Integrify
A workflow diagram demonstrates all the significant steps of a process or business unit. It helps you understand the big picture and relationships between ... 於 www.integrify.com -
#71.3 Types of Flowcharts You Can Use for Workflow Management
Flowcharts are one subset of workflow diagrams, which in themselves are a part of the world of Business Process Modeling (or Business Process ... 於 gravityflow.io -
#72.Process flow diagrams | IST Project Management Office
Process flow diagrams A process model is a formal way of representing how a business operates. A process flow diagram is one method of representing a ... 於 uwaterloo.ca -
#73.How to create an awesome workflow diagram — and why you ...
A workflow diagram is a step-by-step, linear representation of a business process from start to finish. It shows how individual tasks, ... 於 cacoo.com -
#74.Process diagrams - IBM Blueworks Live
Process diagrams · Activities Activities represent the work that is performed in a process. · Events Events represent the things that might happen during a ... 於 www.blueworkslive.com -
#75.Process Flow Diagram Images, Stock Photos & Vectors
Find process flow diagram stock images in HD and millions of other royalty-free stock photos, illustrations and vectors in the Shutterstock collection. 於 www.shutterstock.com -
#76.What Is a Flow Chart? - Leading Edge Group
A flowchart is a visual representation of a process that makes it easy to understand the process at a glance. Flow charts depict the nature and ... 於 www.leadingedgegroup.com -
#77.️ 4 Most Common Types of Flowcharts + Templates - Gliffy
A flowchart is a diagram that shows each step or progression through a process. Lines indicate directional flow and there's a standard set of ... 於 www.gliffy.com -
#78.Flow Diagrams - Axure Docs
You can use Axure RP to make flowcharts, user flows, business process models, and other flow diagrams. Add shape, image, and snapshot widgets to serve as ... 於 docs.axure.com -
#79.Best flowchart software of 2021 | TechRadar
For a simple and easy solution to organize and manage workflows, ideas, and projects, check out our ... Diagram apps for workflow management. 於 www.techradar.com -
#80.How to Design a User Flow Diagram for Your Website
A user flow diagram can help you to nail your content marketing strategy. In this article, we explain what user flow is and how to map it ... 於 optinmonster.com -
#81.Workflow Diagrams | EdrawMax
A workflow diagram is a graphical representation of workflow with the help of a flowchart, which involves the illustration of business processes ... 於 www.edrawsoft.com -
#82.User Flow Diagram Template for FigJam - Figma
This is FigJam version of original User Flow Diagram Template for Figma you can find here: User Flow Diagram Template (Dribbble)Video with instructions ... 於 www.figma.com -
#83.What is a Flowchart? Process Flow Diagrams & Maps | ASQ
A flowchart is a picture of the separate steps of a process in sequential order. It is a generic tool that can be adapted for a wide variety of purposes, and ... 於 asq.org -
#84.Guide to Create a Business Process Diagram - Cflow
Improves productivity: Workflow diagrams can be used to visualize process flow under various scenarios. Process workflow can be adjusted for ... 於 www.cflowapps.com -
#85.Why My Boss won't Draw a Workflow Chart? - Questetra BPM ...
The biggest feature of a Workflow diagram is that it is described graphically, whereas a work manual is written in words. A “figure to know the ... 於 questetra.com -
#86.Flow Charts - Problem-Solving Skills From MindTools.com
Flow charts are simple diagrams that map out a process, so that you can easily communicate it to other people. You can also use them to define and analyze a ... 於 www.mindtools.com -
#87.What is the difference between a data flow diagram and a flow ...
A flow chart details the processes to follow. A DFD details the flow of data through a system. In a flow chart, the arrows represent ... 於 stackoverflow.com -
#88.Process Map for Basic Flowchart - Microsoft templates
A process flow chart template uses symbols and diagrams to depict complex processes from ... Just enter your process data to the Excel flowchart template, ... 於 templates.office.com -
#89.7 Smart Flowchart Maker to Create Process, Diagram and More
For instance, Sterneckert has classified the charts based on “Flow Control”. According to him, flowcharts are of 4 types. They are: Document ... 於 geekflare.com -
#90.How to Make a Workflow Chart From Excel - Small Business ...
Take advantage of this smart move with Excel SmartArt workflow charts. 1. Launch Microsoft Excel. Click the “Insert” tab. Click the “SmartArt ... 於 smallbusiness.chron.com -
#91.Workflow Diagram: What It Is and Why You Need One - Unito
A workflow diagram is a flowchart representing every step of your workflow, including dependencies and responsible parties. It can range from a ... 於 unito.io -
#92.What is a Flow Diagram? | Slickplan
A flow diagram, or flowchart, is a specific type of activity diagram that communicates a sequence of actions or movements within a complex system. 於 slickplan.com -
#93.User Flow Diagrams - FlowMapp
User Flow is a great tool for UX planning. Create user flows, modify diagrams, invite the team to collaborate and share it. Get your free account right now! 於 www.flowmapp.com -
#94.Flow Chart/Process Flow Diagram
A Flow Chart (also known as a Process Flow Diagram or Process Map) is a diagram of the steps in a process and their sequence. Two types of flow charts are ... 於 www.med.unc.edu -
#95.A Complete Guide to Using Workflow Diagrams | MindManager
A workflow diagram is a visual representation of a business process from start to finish. This diagram runs in a linear line and takes you through the workflow ... 於 www.mindmanager.com -
#96.What is a workflow diagram and who uses them? - Monday.com
A workflow diagram, then, is a visual overview of a business process or workflow, most commonly through a flow chart. 於 monday.com -
#97.View Workflow chart - Atlassian Community
How can I return the "View Workflow" link in a Jira issue? When Jira updated, it went missing. 於 community.atlassian.com -
#98.Workflow Diagrams: What They Are, and Where to Use Them
A workflow diagram is a basic visual layout of a business process. Using it, you can represent the various tasks involved, as well as the ... 於 kissflow.com