Tumor scan的問題,我們搜遍了碩博士論文和台灣出版的書籍,推薦Chase, Alexander寫的 10 Lessons I Learned After Battling With Cancer Twice 和中村仁一的 不要把生命寄放在醫生那!-掌握醫療自主權,擁有人生圓滿的終點!都 可以從中找到所需的評價。
這兩本書分別來自 和原水所出版 。
國立臺北科技大學 電資學院外國學生專班(iEECS) 白敦文所指導 VAIBHAV KUMAR SUNKARIA的 An Integrated Approach For Uncovering Novel DNA Methylation Biomarkers For Non-small Cell Lung Carcinoma (2022),提出Tumor scan關鍵因素是什麼,來自於Lung Cancer、LUAD、LUSC、NSCLC、DNA methylation、Comorbidity Disease、Biomarkers、SCT、FOXD3、TRIM58、TAC1。
而第二篇論文慈濟大學 醫學資訊學系碩士班 潘健一所指導 林怡均的 基於影像前處理的卷積神經網路偵測ST段上升型心肌梗塞疾病 (2021),提出因為有 ST段上升型心肌梗塞、心電圖、卷積神經網絡深度學習、影像前處理、紙本心電圖的重點而找出了 Tumor scan的解答。
10 Lessons I Learned After Battling With Cancer Twice
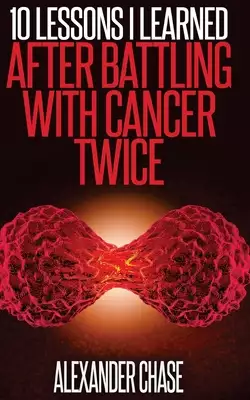
為了解決Tumor scan 的問題,作者Chase, Alexander 這樣論述:
A PERSONAL HISTORY OF MY VERY OWN BATTLE WITH CANCER. 10 LESSONS I LEARNED AFTER BATTLING WITH CANCER TWICE AT THE AGE OF 25. Being diagnosed with cancer can really break you down especially in a case like mine. I have two children that at the time were only 3 and 5 years old, a very difficult si
tuation to explain and quite difficult to understand. I remember waiting in the doctor's room for the report with the results from my last surgery, a report that changed my life and put an end to a very unforgettable experience. People often require a lot of time to process something like this. Some
break down in tears while others go into a state of denial and disbelief. However, sooner or later, everyone comes to terms with it. It is what you do after that realization which is the most important step. I am a survivor, a very proud and happy one, having fought cancer twice before I turned 25
years old. In June 2013 I was diagnosed with cancer. The doctors told me that I had a tumor in the lower abdomen. Thankfully for me, the diagnosis was made on time and the tumor had not grown so much that a simple surgery would do it (as per the doctor). The treatment that followed was pretty straig
ht forward and soon my life returned to normal. However, it wasn't the end of the story for me. Because in March 2014, a scan revealed that I had now a metastasis from the previous cancer, but this time, it targeted the lower lobe of my left lung, measuring about 8 CMs. This revelation really had a
massive impact on me. The doctors did not have an answer, it literally felt like the entire world was dropped on my shoulders. But inside I felt that this new cancer was a result of the poor diet, stress, and my overall lifestyle. Thus I had to go through the process all over again but this time, ch
emotherapies and multiples surgeries were on the way. People who are aware of the effects of chemotherapy would sympathize with me when I say it was the toughest time of my life. But you know what they say, adversity is a great teacher. In this short book, I will share 10 lessons I learned after bat
tling with Cancer twice by the age of 25. Lesson 1 Stop Caring About What Others Think About You. Lesson 2 Develop An Appreciation For Life. Lesson 3 Be Thankful For What You Have Lesson 4 Follow Your Dreams Lesson 5 Your Body Is Your Temple Lesson 6 Live In The Present Lesson 7 Take Responsibility
Lesson 8 Stop Blaming Others Lesson 9 Discover Yourself Lesson 10 Summing It All Up
An Integrated Approach For Uncovering Novel DNA Methylation Biomarkers For Non-small Cell Lung Carcinoma
為了解決Tumor scan 的問題,作者VAIBHAV KUMAR SUNKARIA 這樣論述:
Introduction - Lung cancer is one of primal and ubiquitous cause of cancer related fatalities in the world. Leading cause of these fatalities is non-small cell lung cancer (NSCLC) with a proportion of 85%. The major subtypes of NSCLC are Lung Adenocarcinoma (LUAD) and Lung Small Cell Carcinoma (LUS
C). Early-stage surgical detection and removal of tumor offers a favorable prognosis and better survival rates. However, a major portion of 75% subjects have stage III/IV at the time of diagnosis and despite advanced major developments in oncology survival rates remain poor. Carcinogens produce wide
spread DNA methylation changes within cells. These changes are characterized by globally hyper or hypo methylated regions around CpG islands, many of these changes occur early in tumorigenesis and are highly prevalent across a tumor type.Structure - This research work took advantage of publicly avai
lable methylation profiling resources and relevant comorbidities for lung cancer patients extracted from meta-analysis of scientific review and journal available at PubMed and CNKI search which were combined systematically to explore effective DNA methylation markers for NSCLC. We also tried to iden
tify common CpG loci between Caucasian, Black and Asian racial groups for identifying ubiquitous candidate genes thoroughly. Statistical analysis and GO ontology were also conducted to explore associated novel biomarkers. These novel findings could facilitate design of accurate diagnostic panel for
practical clinical relevance.Methodology - DNA methylation profiles were extracted from TCGA for 418 LUAD and 370 LUSC tissue samples from patients compared with 32 and 42 non-malignant ones respectively. Standard pipeline was conducted to discover significant differentially methylated sites as prim
ary biomarkers. Secondary biomarkers were extracted by incorporating genes associated with comorbidities from meta-analysis of research articles. Concordant candidates were utilized for NSCLC relevant biomarker candidates. Gene ontology annotations were used to calculate gene-pair distance matrix fo
r all candidate biomarkers. Clustering algorithms were utilized to categorize candidate genes into different functional groups using the gene distance matrix. There were 35 CpG loci identified by comparing TCGA training cohort with GEO testing cohort from these functional groups, and 4 gene-based pa
nel was devised after finding highly discriminatory diagnostic panel through combinatorial validation of each functional cluster.Results – To evaluate the gene panel for NSCLC, the methylation levels of SCT(Secritin), FOXD3(Forkhead Box D3), TRIM58(Tripartite Motif Containing 58) and TAC1(Tachikinin
1) were tested. Individually each gene showed significant methylation difference between LUAD and LUSC training cohort. Combined 4-gene panel AUC, sensitivity/specificity were evaluated with 0.9596, 90.43%/100% in LUAD; 0.949, 86.95%/98.21% in LUSC TCGA training cohort; 0.94, 85.92%/97.37 in GEO 66
836; 0.91,89.17%/100% in GEO 83842 smokers; 0.948, 91.67%/100% in GEO83842 non-smokers independent testing cohort. Our study validates SCT, FOXD3, TRIM58 and TAC1 based gene panel has great potential in early recognition of NSCLC undetermined lung nodules. The findings can yield universally accurate
and robust markers facilitating early diagnosis and rapid severity examination.
不要把生命寄放在醫生那!-掌握醫療自主權,擁有人生圓滿的終點!
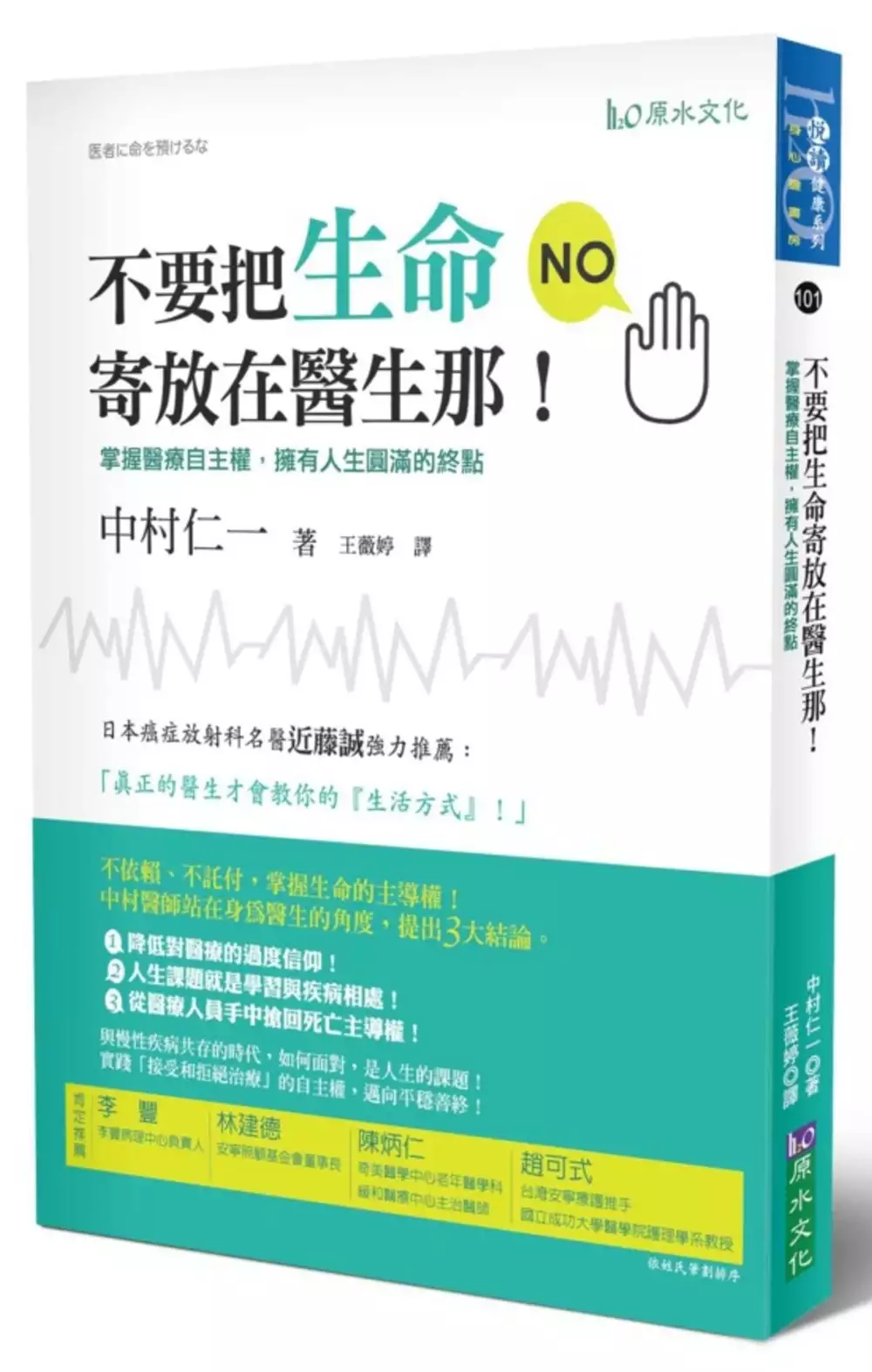
為了解決Tumor scan 的問題,作者中村仁一 這樣論述:
日本癌症放射科名醫近藤誠強力推薦: 「真正的醫生才會教你的『生活方式』!」 面對充滿「不確定性」的醫療,我希望大家不要抱著過度期待,縱使採用最新醫療技術,也未必能康復,因此不要隨便將自己的生命交到醫護人員的手上,而是要善盡自己身為「生命主人」的責任。 ──中村仁一 不依賴、不託付,掌握生命的主導權! 中村醫師站在身為醫生的角度,提出三大結論。 降低對醫療的過度信仰! 人生課題就是學習與疾病相處! 從醫療人員手中搶回死亡主導權! 與慢性疾病共存的時代,如何面對,是人生的課題! 實踐「接受和拒絕治療」的自主權,邁向平穩善終! 你是否對醫
療的實態有所誤解?醫療的進步反而無法平穩善終? 中村醫師道出醫療前線的真相,帶你重新思考及面對生死課題! 醫療人員跟患者的「最好治療方法」不一樣! 真正的名醫,不講專業用語,會為病人著想! 老化無藥可醫,別對醫療有不切實際的幻想! 尊重患者本人意願不要讓積極治療變成折磨! 無視本人意願所進行的醫療行為是一種傷害! 面臨生死問題時,家人未必是最了解患者的! 「告知」是了解臨終患者及需求的重要關鍵! 患者有權利了解並且選擇正確且適合的醫療! 採用最新醫療技術進行治療,也未必能康復! 為了讓當下活得精彩,就必須正視死亡課題! 肯定推薦(依姓氏筆劃排序)
李豐病理中心負責人 李 豐 安寧照顧基金會 林建德 奇美醫學中心老年醫學科、緩和醫療中心主治醫師 陳炳仁 國立成功大學醫學院護理學系教授、台灣安寧療護推手 趙可式
基於影像前處理的卷積神經網路偵測ST段上升型心肌梗塞疾病
為了解決Tumor scan 的問題,作者林怡均 這樣論述:
心血管疾病一直都是國人十大死因的前幾名,其中急性冠心症(Acute Coronary Syndrome, ACS)最為致命。急性冠心症的臨床機轉為供應心臟的冠狀動脈血管產生狹窄、阻塞,使心肌無法獲得氧氣、營養,進而引起心臟壞死,其中又以ST段上升心肌梗塞(STEMI)疾病的心肌受損程度會隨著時間的增加而迅速擴大最為危急。在診斷方面,急性冠心症的主要診斷工具為心電圖,心電圖以非侵入式的方式監測、紀錄下心臟的生理活動並產生心電圖,醫生可根據心電圖去區分急性冠心症的類型,進而決定進行何種治療。現今台灣的救護車多配置生理監視器,在出勤時能針對疑似心臟疾病患者做初步的判斷,在救護途中將量測的心電圖回傳
遠端醫院的醫師進行判斷,這樣的作業模式須依賴心臟專科醫師隨時待命來完成,效率較為低落,若使用科技輔助,將能大幅減少時間成本,達到迅速判讀、準確救護的目的。近年來,由於深度學習方法迅速進展,特別是關於影像分類的CNN模型能夠出色的解決複雜的影像問題,因此被廣泛運用於醫學影像分類。然而一般訓練CNN模型需要大量的影像資料才能獲得準確的分類結果,然而一般醫院的STEMI患者的數量並不算多。本研究的目的在探討心電圖資料相對較少的前提下,分析不同的影像前處理方法對CNN為基礎的深度學習模型的表現,包含影像去背、形態學處理、影像增強等影像前處理技術優化心電圖影像,最後再透過不同的CNN模型,判斷ST段上升
型心肌梗塞患者。本研究中,我們僅使用602張圖片,分別在多個CNN模型中進行訓練、測試,包含EfficientNet、ResNet、DenseNet皆得到87%以上的準確率,證實影像前處理之重要性。