Multi link Operation的問題,透過圖書和論文來找解法和答案更準確安心。 我們找到下列懶人包和總整理
Multi link Operation的問題,我們搜遍了碩博士論文和台灣出版的書籍,推薦黃國源寫的 類神經網路(第四版)(附範例光碟) 和黃國源的 類神經網路(第二版)(附範例光碟)都 可以從中找到所需的評價。
另外網站全球第一!聯發科大秀Wi-Fi 7技術首批終端產品2023年上市也說明:聯發科驗證了其Filogic Wi-Fi 7技術可達到IEEE 802.11be定義的最大速度。同時,聯發科還展示了多重連接模式技術(Multi-Link Operation,MLO),可同時 ...
這兩本書分別來自全華圖書 和全華圖書所出版 。
元智大學 電機工程學系甲組 林鴻文、彭朋瑞所指導 王崴弘的 具有5標記前饋式等化器之80 Gb/s不歸零傳送機於四十奈米CMOS製程 (2021),提出Multi link Operation關鍵因素是什麼,來自於電流模式驅動器、前饋式等化器、不歸零、傳送機。
而第二篇論文國立雲林科技大學 工業工程與管理系 駱景堯所指導 儲玉瑄的 應用機器學習於永磁同步馬達轉子溫度預測之研究 (2021),提出因為有 PMSM、機器學習、轉子溫度、迴歸分析的重點而找出了 Multi link Operation的解答。
最後網站聯發科完成業界首家Wi-Fi 7 技術現場展示,終端產品2023 年上市則補充:聯發科驗證Filogic Wi-Fi 7 技術可達IEEE 802.11be 定義的最大速度,還展示多重連接模式技術(Multi-Link Operation,MLO),可同時聚合不同頻段上的 ...
類神經網路(第四版)(附範例光碟)
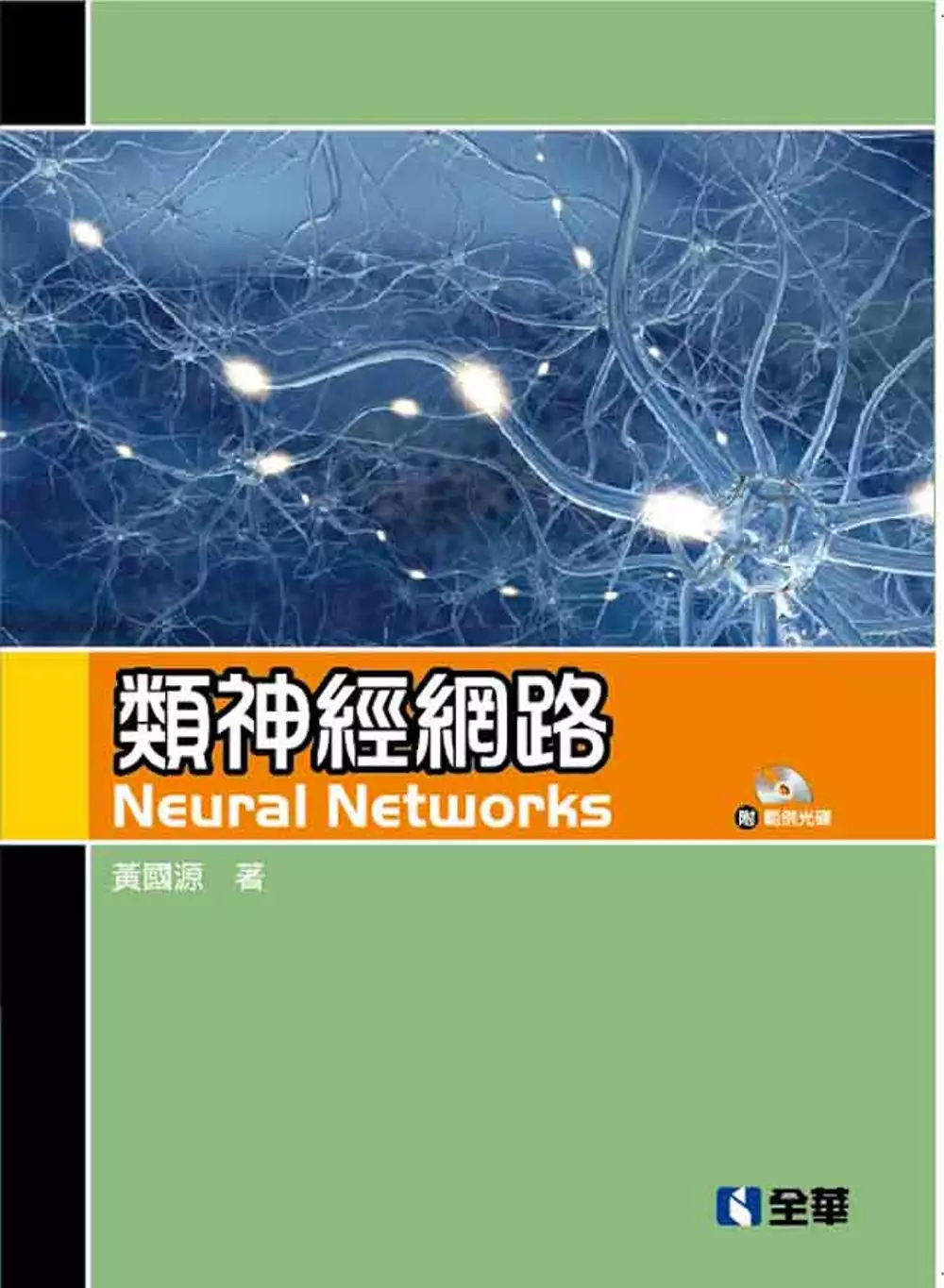
為了解決Multi link Operation 的問題,作者黃國源 這樣論述:
人類的頭腦約由1011個神經元所組成,所有的訊息就在神經元與神經元間靠著軸突及樹突的發送與接收來傳遞。在這樣的一個過程中,所接收進來的各種訊息被分類或辨認,進而形成了人類的認知與思維。現在我們利用數學的計算來模擬神經元的運作,進而模擬神經網路的傳送,以期達到分類或辨認。類神經網路的特點為學習,學習的目的是要調整神經腱的大小,即調整加權係數,我們要探討各種就是學習法則。的類神經網路的模型及其加權係數的調整公式,也本書著重於利用類神經網路的方法於圖形辨識與最佳化問題之解決,因此將先介紹傳統的識別方法,再介紹類神經網路的各種理論及模型。本書提供基本的例子讓讀者容易了解,容易進入類神經網路的領域,
在探討的多個模型中,均有自己提出的見解。 本書特色 1.本書著重於利用類神經網路的方法於模式辨別與最佳化問題之解決。 2.提供基礎範例讓讀者容易了解,容易進入類神經網路的領域。 3.在何普菲模型應用於解銷售員旅行問題(TSP)走最短距離的迴旋距離的優化,有詳細的分析;在何普菲類神經網路及一般化的蜂窩神經網絡也有做基本的介紹。 第一章 簡介 1.1 圖型的定義與圖型識別的方法 1.2 Decision-theoretic Approach的圖形識別與空間分割 1.3 Pattern Recognition Systems 1.4 Non-parametric & Para
metric Methods 1.5 人類頭腦的Neuron與模擬的Perceptron 1.6 Two Class Data分佈的複雜性 1.7 Activation Function 1.8 Development History of Neural Networks 1.9 Neural Network Applications 第二章 DECISION-THEORETIC PATTERN RECOGNITION 決策理論的圖形識別Decision-theoretic Approach的圖形識別與Discriminant Functions 2.2 Nonparametric Patt
ern Recognition非參數式之圖形識別:Using Discriminant Functions 2.2.1 Linear discriminant functions for pattern recognition 2.2.2 Nonlinear discriminant functions for pattern recognition 2.2.3 Perpendicular bisector 2.2.4 Minimum-distance classifier 2.2.5 Minimum-distance classifier with respect to point sets
(Piecewise-linear discriminant functions, Nearest-neighbor classification) 2.2.6 N-nearest neighbor classification rule 2.3 Parametric Pattern Recognition 參數式之圖形識別 2.3.1 Bayes theorem (貝氏定理) and probability density function (pdf) 2.3.2 Bayes (Parametric) classification rule (貝氏分類法則) 2.3.3 Sequentia
l classification 2.3.4 Neyman-Pearson test 2.3.5 Linear Classifier Design 2.3.6 Feature selection 2.3.7 Error estimation 2.4 Unsupervised Pattern Recognition 2.4.1 Minimum spanning tree (MST) clustering 2.4.2 K-means clustering 2.4.3 Hierarchical Clustering Using Dendrogram (Unsupervised Clustering)
2 第三章 PERCEPTRON 認知器數學上解Decision Boundary之困難 3.2 Perceptron 3.3 Classification 3.4 Training (Learning) 3.5 Flowcharts of Perceptron 3.6 Convergence Proof of Perceptron for Fixed Increment Training Procedure 3.7 Perceptron for Logic Operation 3.8 Layered Machine (Committee Machine/Voting Machine) 3
.9 Multiclass Perceptrons 3.10 Perceptron with Sigmoidal Activation Function and Learning by Gradient Descent Method 3.11 Modified Fixed-increment Training Procedure 3.12 Multiclass Perceptron with Delta Learning Rule 3.13 Widrow-Hoff Learning Rule 3.14 Correlation Learning Rule 第四章 MULTILAYER PERC
EPTRON 多層認知器 Introduction 4.2 設計Multilayer Perceptron with 1 Hidden Layer 解XOR的分類問題 4.3 Gradient and Gradient Descent Method in Optimization 4.4 Multilayer Perceptron (MLP) and Forward Computation 4.5 Back-propagation Learning Rule (BP) 4.5.1 Analysis 4.5.2 Back-propagation learning algorithm of one
-hidden layer perceptron (I) 4.5.3 Back-propagation learning algorithm of one-hidden layer perceptron (II) 4.6 Experiment of XOR Classification & Discussions 4.7 On Hidden Nodes for Neural Nets 4.8 Application - NETtalk:A Parallel Network That Learns to Read Aloud 4.9 Functional-Link Net 第五章 RADIAL
BASIS FUNCTION NETWORK (RBF) 輻射基底函數網路 Introduction 5.2 RBF Network 第一層的Learning Algorithm 5.3 RBF Network 第二層的Learning Algorithm 5.4 設計RBF Model to Classify XOR Patterns 第六章 SUPPORT VECTOR MACHINE (SVM) 支持向量的分類器Introduction 6.2 點到Hyperplane之距離 6.3 Role of Support Vectors in Optimal Margin Classifi
er for Linearly Separable Case 6.4 Find Optimal Margin Classifier for Linearly Separable Case 6.5 SVM for Nonseparable Patterns 6.5.1 Primal Problem 6.5.2 Dual Problem 6.6 Feature Transformation and Support Vector Machine (SVM) – Kernel SVM 6.6.1 Primal Problem and Optimal Separating Hyperplane之建立 6
.6.2 在Dual Problem上求解新的Feature Space上的Support Vector Machine 6.6.3 Gradient Ascent的調適性的方法求 Lagrange Multipliers 6.7 Multiclss Classification Using Support Vector Machine 6.7.1 Maximum Selection Classification System Using SVMs 6.7.2 利用SVM 於數字辨識的樹狀分類系統 (Tree Classification System) 6.7.3 Multi-class C
lassification Using Many Binary SVMs 6.8 SVM Examples 6.8.1 直接利用Lagrange method (沒有利用KKT conditions 的Lagrange method) 6.8.2 利用加入KKT 的Lagrange method 6.8.3 Support Vector Machine (SVM) Using Feature Transformation – Kernel SVM 6.8 Exercise 第七章 KOHONEN’S SELF-ORGANIZING NEURAL NET 自我組織的類神經網路 Winner-T
ake-All Learning Rule 7.2 Kohonen’s Self-organizing Feature Maps 7.3 Self-organizing Feature Maps於TSP 第八章 PRINCIPAL COMPONENT NEURAL NET 主分量類神經網路Introduction 8.2 Hebbian Learning Rule 8.3 Oja的學習法則 8.4 Neural Network of Generalized Hebbian Learning Rule 8.5 Data Compression 8.6 Effect of Adding One
Extra Point along the Direction of Existing Eigenvector 8.7 Neural network的PCA的應用 第九章 HOPFIELD NEURAL NET 9.1 Lyapunov Function 9.2 Discrete Hopfield Model 9.3 Analog Hopfield Model 9.3.1 Circuits and Power 9.3.2 Analog Hopfield Model 9.4 Optimization Application of Hopfield Model to TSP 9.5 與Hopfi
eld Neural Net有關的研究與應用 第十章 CELLULAR NEURAL NETWORK 蜂巢式類神經網路 10.1 簡介 10.2 蜂巢式類神經網路架構 10.3 蜂巢式類神經網路的穩定性分析 10.4 蜂巢式類神經網路與Hopfield神經網路的比較 10.5 離散蜂巢式類神經網路 第十一章 HAMMING NET 11.1 Introduction 11.2 Hamming Distance and Matching Score 11.3 Hamming Net Algorithm 11.4 Comparator 第十二章 ADAPTIVE RESONANCE THEO
RY NET (ART) 12.1 Introduction 12.2 ART1 Neural Model 12.3 Carpenter/Grossberg ART1 Net的Algorithm 12.4 Revised ART algorithm 第十三章 FUZZY, CLUSTERING, AND NEURAL NETWORKS 13.1 Fuzzy C-means Clustering Algorithm 13.2 Fuzzy Perceptron 13.3 Pocket Learning Algorithm 13.4 Fuzzy Pocket 參考文獻 附錄 Appendix
A:Inner Product (內積) Appendix B:Line Property and Distance from Point to Line Appendix C:Covariance Matrix Appendix D:Gram–Schmidt Orthonormal Procedure Appendix E:Lagrange Multipliers Method Appendix F:Gradient, Gradient Descent and Ascent Methods in Optimization Appendix G:Derivation of Oja’s lear
ning rule Appendix H:類神經網路程式實驗報告範例 Appendix I:實驗報告範例之電腦程式 Appendix J:MATLAB Program of Perceptron Appendix K:MATLAB Program of Multilayer Perceptron Appendix L:FORTRAN Program for Perceptron Appendix M:畫aX+bY+cZ+常數= 0的平面的Matlab電腦程式 Appendix N:Support Vector Machine的數學推導 Appendix O:Projects Appendix
P:Project #1的部份Matlab程式
Multi link Operation進入發燒排行的影片
飛利浦抗敏清淨除濕機DE5205是功夫家庭的秘密武器
Our Kungfu family has a secret weapon to share with you all—
幫助我們日常打怪而且全年無休!
It helps us fight monsters daily and does not rest!
平時忙著導演編劇工作,武術指導,戲劇學校營運的功夫家庭,健康是最重要的大事,空氣品質尤其重視!一向以 teamwork 方式共同承擔家務的我們:功夫爸爸負責清水箱,功夫媽媽負責清洗濾網,空氣清淨指數則交給 Kali看就行了(看著飛利浦先生身上的圈圈,都要blue才是好空氣歐,其他顏色都no no)
For our Kungfu family, usually busy with acting, directing, screenwriting, teaching martial arts, and running an actor’s studio, health is the most important thing; and air quality is at the top of that list! As a martial arts family, we always do everything as a team: Kungfu poppa is responsible for the cleaning the water tank, Kungfu Momma is responsible for cleaning the filter, and the air purification index is left to Kali. (She checks out the circle on Mr. Philips: blue is clean air and other colors are a no-no!)
自從用了飛利浦 #DE5205 (諧音我愛你我)一台搞定3件事,多功能的原廠設定 #抗敏 #清淨 #除濕 好簡單! 大幅改善生活品質,讓住在山區的我們超級仰賴它!有了二寶之後,家裡的溼度和空氣品質真的要注意,我們更把 #飛利浦抗敏清淨除濕機 帶去月子中心再帶回來,根本是生活中的 Must啊!推薦所有有寶寶的家庭可以入手一台!(兩台也可以!)
Ever since we used Philips #DE5205 (the model number is Chinese homonym for I love you and me together), it simplifies everything, it’s multi-functional factory settings are 3 in 1. #preventsmold #purifiestheair #dehumidifies Living in the mountains, it totally transformed our or life and we rely on it so much! After having the second baby, we really need to be more cautious, we even took #Phillips to the postpartum center and then brought it back. It definitely is a must-have in our household! It is highly recommended that you get one (or two) if you have babies!
用影片及文字幫大家整理一下 #DE5205的優勢,請看!
Check our video to learn about the advantages of #DE5205.
高濾除、高效能、高安全 It’s high-filtration, high-efficiency, high-safety
1. 奈米級0.02濾淨力:抗敏級HEPA濾網,德國IUTA認證可有效濾除至最小PM0.02細懸浮粒子,提升空氣品質
Nano-level 0.02 Filtration: Anti-allergic HEPA filter, German IUTA certified, which can effectively filter down to the smallest PM0.02 fine suspended particles, and improves air quality
2. 大容量除溼:每日13 公升大除溼力
Large capacity dehumidifier: 13 liters water capacity per day
3. 四種智能模式:舒適濕度/連續除濕/乾衣模式/空氣淨化,按照需求變換功能
Four smart modes: comfortable humidity/continuous dehumidification/drying mode/air purification, changes functions according to needs
4.一級節能標準:節能再退稅 $1,200
Level 1 Energy Conservation Standard: Energy-saving and tax rebate $1,200
功夫爸爸最愛:水箱提把,方便好提不漏水(當作是微量級健身)
Kungfu Poppa’s favorite: the water tank handle, which is convenient and easy to carry and does not leak (as a micro-level fitness)
功夫媽媽最愛:底部滾輪,白天移到遊戲間超方便
Kungfu Momma’s favorite: the bottom scroll wheel is super convenient to move to the game room during the day
功夫大寶最愛:空氣品質燈,因為看到藍燈就是保護了妹妹
Kungfu Big Baby’s favorite: air quality light, because seeing the blue light is to protect her sister
功夫小寶最愛:低噪音運轉,才29分貝耶!讓我可以安心呼呼大睡
Kungfu Little Baby’s favorite: low noise operation, only 29 decibels! So she can sleep peacefully
立即購買還享有兩年全球保固喔
Click the link below to get a free two-year worldwide warranty:
https://reurl.cc/0Dbd7M
如果想到櫃上實際看看的話可以參考以下連結
Or go directly to the store to check it out here:
https://www.store-philips.tw/v2/Shop/StoreList/1956
#Philips #飛利浦 #抗敏清淨除濕機 #DE5205 #三重功效 #抗敏 #清淨 #除濕
具有5標記前饋式等化器之80 Gb/s不歸零傳送機於四十奈米CMOS製程
為了解決Multi link Operation 的問題,作者王崴弘 這樣論述:
本論文提出一個有5標記前饋式等化器的80Gb/s的傳送機,驅動器使用的是電流模式的架構,粗調及微調的機制在精細度及輸出頻寬中達到平衡。80-Gb/s的2:1多工器利用四分之一速率時脈取樣在資料轉態點的方式來降低時脈所需的功率消耗,相位內插器包含在時脈路徑中,用於調整前一級多工器的轉態點位置,從而最大限度的減少80Gb/s輸出資料的週期性抖動。 傳送機皆採用40奈米製程做設計及製造,此傳送機達到80-Gb/s的速度,功率消耗為650mW於1V/1.2V電源電壓下測試,再經過5標記前饋式等化器的粗調細調補償後,此傳送機在80-Gb/s的速度輸出眼開為50mV。
類神經網路(第二版)(附範例光碟)
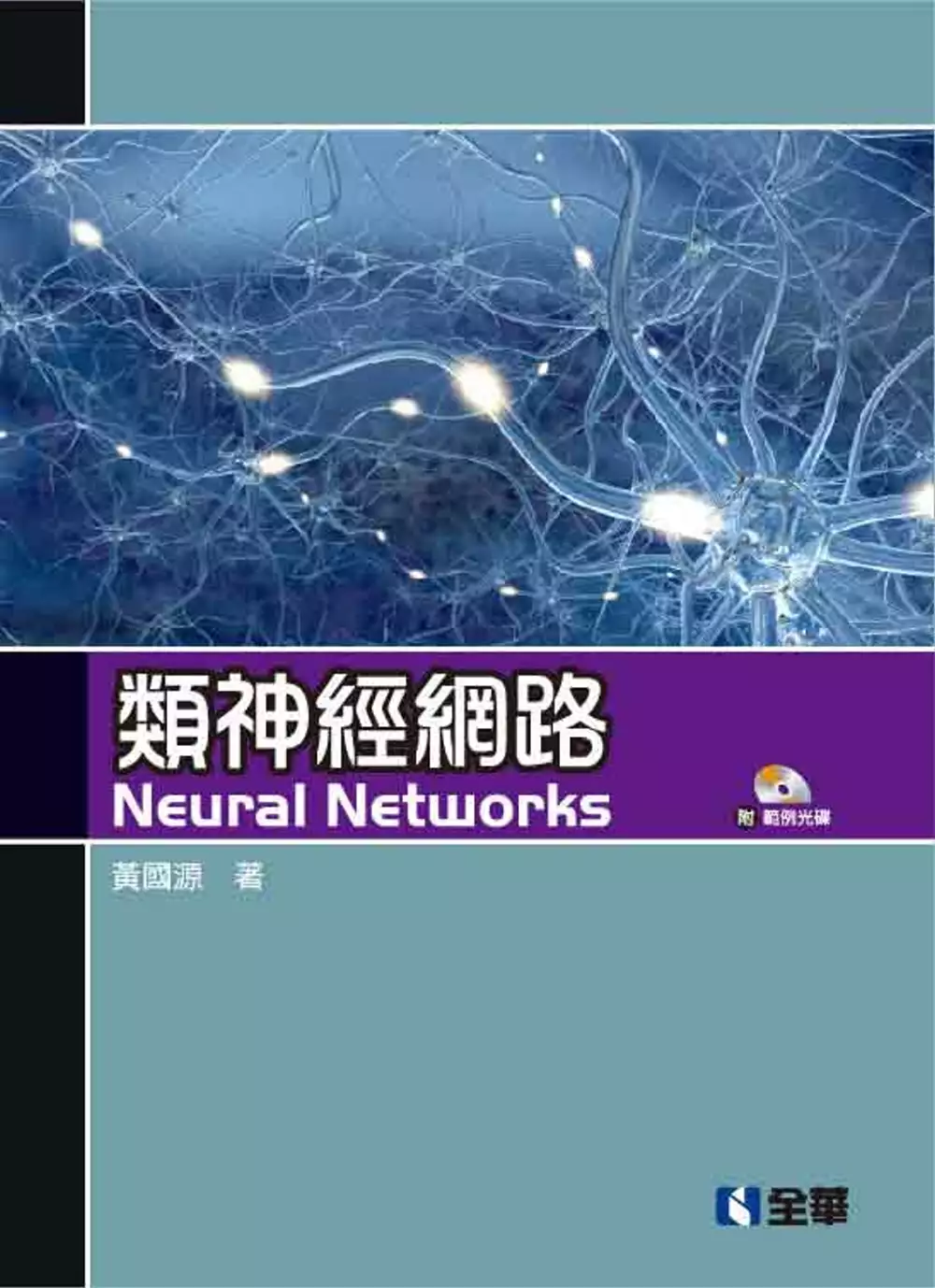
為了解決Multi link Operation 的問題,作者黃國源 這樣論述:
人類的頭腦約由1011 個神經元所組成,所有的訊息就在神經元與神經元間靠著軸突及樹突的發送與接收來傳遞。在這樣的一個過程中,所接收進來的各種訊息被分類或辨認,進而形成了人類的認知與思維。現在我們利用數學的計算來模擬神經元的運作,進而模擬神經網路的傳送,以期達到分類或辨認。類神經網路的特點為學習,學習的目的是要調整神經腱的大小,即調整加權係數,我們要探討各種就是學習法則。的類神經網路的模型及其加權係數的調整公式,也本書著重於利用類神經網路的方法於圖形辨識與最佳化問題之解決,因此將先介紹傳統的識別方法,再介紹類神經網路的各種理論及模型。本書提供基本的例子讓讀者容易了解,容易進入類神經網路的領域
,在探討的多個模型中,均有自己提出的見解。 本書特色 1.本書著重於利用類神經網路的方法於模式辨別與最佳化問題之解決。 2.提供基礎範例讓讀者容易了解,容易進入類神經網路的領域。 3.在何普菲模型應用於解銷售員旅行問題(TSP) 走最短距離的迴旋距離的優化,有詳細的分析;在何普菲類神經網路及一般化的蜂窩神經網絡也有做基本的介紹。 第一章 簡介 1.1 圖型的定義與圖型識別的方法 1.2 Decision-theoretic Approach的圖形識別與空間分割 1.3 Pattern Recognition Systems 1.4 Non-parametric &
Parametric Methods 1.5 人類頭腦的Neuron與模擬的Perceptron 1.6 Two Class Data分佈的複雜性 1.7 Activation Function 1.8 Development History of Neural Networks 1.9 Neural Network Applications 第二章 DECISION-THEORETIC PATTERN RECOGNITION 決策理論的圖形識別 Decision-theoretic Approach的圖形識別與Discriminant Functions 2.2 Nonparametric
Pattern Recognition非參數式之圖形識別: Using Discriminant Functions 2.2.1 Linear discriminant functions for pattern recognition 2.2.2 Nonlinear discriminant functions for pattern recognition 2.2.3 Perpendicular bisector 2.2.4 Minimum-distance classifier 2.2.5 Minimum-distance classifier with respect to poin
t sets (Piecewise-linear discriminant functions, Nearest-neighbor classification) 2.2.6 N-nearest neighbor classification rule 2.3 Parametric Pattern Recognition 參數式之圖形識別 2.3.1 Bayes theorem (貝氏定理) and probability density function (pdf) 2.3.2 Bayes (Parametric) classification rule (貝氏分類法則) 2.3.3 Seq
uential classification 2.3.4 Neyman-Pearson test 2.3.5 Linear Classifier Design 2.3.6 Feature selection 2.3.7 Error estimation 2.4 Unsupervised Pattern Recognition 2.4.1 Minimum spanning tree (MST) clustering 2.4.2 K-means clustering 2.4.3 Hierarchical Clustering Using Dendrogram (Unsupervised Clust
ering) 2 第三章 PERCEPTRON 認知器數學上解Decision Boundary之困難 3.2 Perceptron 3.3 Classification 3.4 Training (Learning) 3.5 Flowcharts of Perceptron 3.6 Convergence Proof of Perceptron for Fixed Increment Training Procedure 3.7 Perceptron for Logic Operation 3.8 Layered Machine (Committee Machine/Voting Mach
ine) 3.9 Multiclass Perceptrons 3.10 Perceptron with Sigmoidal Activation Function and Learning by Gradient Descent Method 3.11 Modified Fixed-increment Training Procedure 3.12 Multiclass Perceptron with Delta Learning Rule 3.13 Widrow-Hoff Learning Rule 3.14 Correlation Learning Rule 第四章 MULTILAYE
R PERCEPTRON 多層認知器 Introduction 4.2 設計Multilayer Perceptron with 1 Hidden Layer 解XOR的分類問題 4.3 Gradient and Gradient Descent Method in Optimization 4.4 Multilayer Perceptron (MLP) and Forward Computation 4.5 Back-propagation Learning Rule (BP) 4.5.1 Analysis 4.5.2 Back-propagation learning algorithm
of one-hidden layer perceptron (I) 4.5.3 Back-propagation learning algorithm of one-hidden layer perceptron (II) 4.6 Experiment of XOR Classification & Discussions 4.7 On Hidden Nodes for Neural Nets 4.8 Application - NETtalk:A Parallel Network That Learns to Read Aloud 4.9 Functional-Link Net 第五章
RADIAL BASIS FUNCTION NETWORK (RBF) 輻射基底函數網路 Introduction 5.2 RBF Network 第一層的Learning Algorithm 5.3 RBF Network 第二層的Learning Algorithm 5.4 設計RBF Model to Classify XOR Patterns 第六章 SUPPORT VECTOR MACHINE (SVM) 支持向量的分類器Introduction 6.2 點到Hyperplane之距離 6.3 Role of Support Vectors in Optimal Margin Cl
assifier for Linearly Separable Case 6.4 Find Optimal Margin Classifier for Linearly Separable Case 6.5 SVM for Nonseparable Patterns 6.5.1 Primal Problem 6.5.2 Dual Problem 6.6 Feature Transformation and Support Vector Machine (SVM) – Kernel SVM 6.6.1 Primal Problem and Optimal Separating Hyperplan
e之建立 6.6.2 在Dual Problem上求解新的Feature Space上的Support Vector Machine 6.6.3 Gradient Ascent的調適性的方法求 Lagrange Multipliers 6.7 Multiclss Classification Using Support Vector Machine 6.7.1 Maximum Selection Classification System Using SVMs 6.7.2 利用SVM 於數字辨識的樹狀分類系統 (Tree Classification System) 6.7.3 Multi-c
lass Classification Using Many Binary SVMs 6.8 SVM Examples 6.8.1 直接利用Lagrange method (沒有利用KKT conditions 的Lagrange method) 6.8.2 利用加入KKT 的Lagrange method 6.8.3 Support Vector Machine (SVM) Using Feature Transformation – Kernel SVM 6.8 Exercise 第七章 KOHONEN’S SELF-ORGANIZING NEURAL NET 自我組織的類神經網路 Wi
nner-Take-All Learning Rule 7.2 Kohonen’s Self-organizing Feature Maps 7.3 Self-organizing Feature Maps於TSP 第八章 PRINCIPAL COMPONENT NEURAL NET 主分量類神經網路Introduction 8.2 Hebbian Learning Rule 8.3 Oja的學習法則 8.4 Neural Network of Generalized Hebbian Learning Rule 8.5 Data Compression 8.6 Effect of Addin
g One Extra Point along the Direction of Existing Eigenvector 8.7 Neural network的PCA的應用 第九章 HOPFIELD NEURAL NET 9.1 Lyapunov Function 9.2 Discrete Hopfield Model 9.3 Analog Hopfield Model 9.3.1 Circuits and Power 9.3.2 Analog Hopfield Model 9.4 Optimization Application of Hopfield Model to TSP 9.5
與Hopfield Neural Net有關的研究與應用 第十章 CELLULAR NEURAL NETWORK 蜂巢式類神經網路 10.1 簡介 10.2 蜂巢式類神經網路架構 10.3 蜂巢式類神經網路的穩定性分析 10.4 蜂巢式類神經網路與Hopfield神經網路的比較 10.5 離散蜂巢式類神經網路 第十一章 HAMMING NET 11.1 Introduction 11.2 Hamming Distance and Matching Score 11.3 Hamming Net Algorithm 11.4 Comparator 第十二章 ADAPTIVE RESONANC
E THEORY NET (ART) 12.1 Introduction 12.2 ART1 Neural Model 12.3 Carpenter/Grossberg ART1 Net的Algorithm 12.4 Revised ART algorithm 第十三章 FUZZY, CLUSTERING, AND NEURAL NETWORKS 13.1 Fuzzy C-means Clustering Algorithm 13.2 Fuzzy Perceptron 13.3 Pocket Learning Algorithm 13.4 Fuzzy Pocket 參考文獻 附錄 Appe
ndix A:Inner Product (內積) Appendix B:Line Property and Distance from Point to Line Appendix C:Covariance Matrix Appendix D:Gram–Schmidt Orthonormal Procedure Appendix E:Lagrange Multipliers Method Appendix F:Gradient, Gradient Descent and Ascent Methods in Optimization Appendix G:Derivation of Oja’s
learning rule Appendix H:類神經網路程式實驗報告範例 Appendix I:實驗報告範例之電腦程式 Appendix J:MATLAB Program of Perceptron Appendix K:MATLAB Program of Multilayer Perceptron Appendix L:FORTRAN Program for Perceptron Appendix M:畫aX+bY+cZ+常數= 0的平面的Matlab電腦程式 Appendix N:Support Vector Machine的數學推導
應用機器學習於永磁同步馬達轉子溫度預測之研究
為了解決Multi link Operation 的問題,作者儲玉瑄 這樣論述:
工業4.0自動化產業興盛,電動車產業為現代主要趨勢,則多數廠商配置永磁同步馬達(PMSM)作為汽車的核心驅動系統,當驅動馬達時會因轉子溫度變化而影響系統效能,如何有效控制溫度變化,實現馬達高效率控制策略,確保PMSM於安全運作與最大使用率的狀態,可降低內部零組件的壽命耗損和提升整體運轉效率。 本研究使用Kaggle提供的PMSM溫度資料集的轉子溫度作為主要探討,因此欲透過傳統迴歸分析與機器學習方法之模型對轉子溫度進行預測,分別使用貝氏嶺迴歸、隨機森林、XGBoost及LightGBM模型,並將上述各預測方法比較之各模型績效。經由各預測方法比較之各模型績效後,得知最佳預測模型為XGBoo
st模型,以利未來將本研究提供於電動車產業配置PMSM的研發與技術,能施以預測性維護馬達溫度狀態,進而防止關鍵性設備故障與停機。
想知道Multi link Operation更多一定要看下面主題
Multi link Operation的網路口碑排行榜
-
#1.Wi-Fi 7 Specifications and features - RF Page
Multi -link technology offers low latency service ... In the current wireless system, access points supports three channels, one lower band 2.4GHz ... 於 www.rfpage.com -
#2.multi-link - 头条搜索
Subsequently, the reporters did not ask the multi-link on the woman. ... MLO:Multi-link Operation MLO 是WiFi 7 上的一个很有趣的技术。 於 m.toutiao.com -
#3.全球第一!聯發科大秀Wi-Fi 7技術首批終端產品2023年上市
聯發科驗證了其Filogic Wi-Fi 7技術可達到IEEE 802.11be定義的最大速度。同時,聯發科還展示了多重連接模式技術(Multi-Link Operation,MLO),可同時 ... 於 finance.ettoday.net -
#4.聯發科完成業界首家Wi-Fi 7 技術現場展示,終端產品2023 年上市
聯發科驗證Filogic Wi-Fi 7 技術可達IEEE 802.11be 定義的最大速度,還展示多重連接模式技術(Multi-Link Operation,MLO),可同時聚合不同頻段上的 ... 於 technews.tw -
#5.聯發科展示Wi-Fi 7技術 - 新通訊
聯發科驗證其Filogic Wi-Fi 7技術可達到IEEE 802.11be定義的最大速度。同時,該公司還展示多重連接模式技術(Multi-Link Operation, MLO),可同時 ... 於 www.2cm.com.tw -
#6.聯發科秀WiFi 7技術終端產品2023年上市 - 民眾日報
聯發科表示,公司技術驗證其Filogic Wi-Fi 7技術可達到IEEE 802.11be定義的最大速度。同時展示了多重連接模式技術(Multi-Link Operation,MLO),可同時 ... 於 www.mypeoplevol.com -
#7.Double Pint Multi-Link Presses-Precision Power ... - EZB2B
It brings revolutionary break through in link presses operation. ○Unique multi-links structure not only completes advantage of link presses but also high ... 於 www.ezb2b.com -
#8.Wi-Fi 7 Explained: Everything to Know About Tomorrow's ...
Additionally, Wi-Fi 7 will introduce Multi-Link Operation (MLO) technology, which allows devices to simultaneously send and receive data ... 於 www.pcmag.com -
#9.Wi-Fi 7 needs refinement to enable network infrastructure ...
These other features will also contribute to lower latency and greater reliability. Multi-Link Operation allows a client device to be connected ... 於 blogs.cisco.com -
#10.multi-link rear - Linguee | 中英词典(更多其他语言)
大量翻译例句关于"multi-link rear" – 英中词典以及8百万条中文译文例句搜索。 於 cn.linguee.com -
#11.Wi-Fi 7(802.11be)前瞻4:MLO(Multi-Link Operation) - 知乎
序言目前802.11be的协议版本已经跨过了draft 1.0,按照协议组的计划应该是先定PHY,然后再定MAC。在802.11be中,PHY一个比较重要的特性就是MLD,即Multi-link Device, ... 於 zhuanlan.zhihu.com -
#12.Pushing the limits of Wi-Fi performance with Wi-Fi 7 - Qualcomm
The highest performance option is High Band Simultaneous Multi-Link, as shown in Fig. 2. The device uses each band as it becomes available and ... 於 www.qualcomm.com -
#13.Wi-Fi 7 Explained: A Solid Upgrade vs 6/E - Dong Knows Tech
Multi -Link Operation or MLO is the most exciting and promising feature of Wi-Fi 7. In a nutshell, MLO is Wi-Fi band aggregation. Like Link ... 於 dongknows.com -
#14.分享預計2024 年才會商用化的Wi-Fi 7 技術特質#802.11ab ...
照片中提到了Multi-link Operation (MLO)、Dala、MLO enables. △ MLO 可結合不同頻段、頻道與AP 提升傳輸速度與降低延遲. 3、加入對Multi-Link ... 於 www.cool3c.com -
#15.Evolution to Wi-Fi 6E and Wi-Fi 7 - ztetechnologies
—Supports 4096 QAM, improves performance by 20% compared with 1024 QAM, and enhances the MIMO mechanism. —Adopts multi-link operation (MLO) and ... 於 www.zte.com.cn -
#16.Wi-Fi 7 Chips Ahoy | Google 'Gone Downhill Fast' | Real-World ...
[And it] introduces a technology called Multi-Link Operation (MLO), which enables devices to aggregate channels and rapidly switch between ... 於 devops.com -
#17.Multi-link Operation in IEEE 802.11be WLANs | DeepAI
The multi-link operation (MLO) is a new feature proposed to be part of the IEEE 802.11be Extremely High Throughput (EHT) amendment. 於 deepai.org -
#18.Multi-Link-Operation in Wi-Fi 7 - Golem.de
WLAN nach 802.11be alias Wi-Fi 7 soll theoretisch über 40 GBit/s erreichen. Zudem werden das 6-GHz-Band und Multi-Link verpflichtend. - Multi-Link-Operation ... 於 www.golem.de -
#19.Qualcomm FastConnect 7800: Introducing Wi-Fi 7 to Power ...
Qualcomm's FastConnect 7800 is a system-level solution featuring 4-stream High Band Simultaneous (HBS) Multi-Link Operations (MLO), ... 於 www.counterpointresearch.com -
#20.聯發科全球首家WiFi 7技術展示聯手韓廠2023年上市 - 翻爆
聯發科技驗證了其Filogic Wi-Fi 7技術可達到IEEE 802.11be定義的最大速度。同時,該公司還展示了多重連接模式技術(Multi-Link Operation,MLO) ... 於 turnnewsapp.com -
#21.Qualcomm unveils Wi-Fi 7 platforms for access points and ...
Shortly put, it will bring Multi-Link Operation (MLO) for utilizing multiple radio links on different channel frequencies. 於 www.gsmarena.com -
#22.聯發科Wi-Fi 7報到! 明年搶市打入韓國電信網通廠 - 蘋果日報
聯發科驗證了其Filogic Wi-Fi 7技術可達到IEEE 802.11be定義的最大速度,更展示了多重連接模式技術(Multi-Link Operation,MLO),可同時聚合不同 ... 於 www.appledaily.com.tw -
#23.Ilya Levitsky - Google Scholar
IITP RAS - Cited by 179 - Wi-Fi networks - multiple channel access and scheduling - non-orthogonal multiple access - multi-link operation 於 scholar.google.com -
#24.Wi-Fi 7 Multi-Link Operation (MLO) white paper | MediaTek (en)
The MediaTek Filogic 880 for access points, routers, repeaters and Mesh networking equipment includes single-chip MAC MLO (Multi-link operation). This unique ... 於 www.mediatek.com -
#25.WIFI EVOLUTION
Multi -Link Operation: The 802.11be target for efficient operations in all available bands, 2.4, 5. & 6 GHz, for load balancing, aggregation of multi-band, ... 於 dione.lib.unipi.gr -
#26.1. wo2021251901 - WIPO Patentscope
COMMUNICATION APPARATUS AND COMMUNICATION METHOD FOR MULTI-LINK TRAFFIC ... the AP comprising: circuitry, which in operation, generates a ... 於 patentscope.wipo.int -
#27.LitePoint Unveils New Test System for WiFi 7 - The Fast Mode
Combining these capabilities with the new multi-link modes, Wi-Fi 7 will power ... 4K QAM modulation, up to 16 MIMO streams, and multi-link operation (MLO). 於 www.thefastmode.com -
#28.更高速的Wi-Fi 7世界要來了?!英特爾提供業界無與倫比的連線 ...
l 支援Multi-link Operation多重連接模式,STA能夠同時透過不同頻段或不同頻道與AP連結,進一步提升最大傳輸速度(多個頻段∕多個頻道同時傳輸)、可靠性(多個頻段∕ ... 於 www.computerdiy.com.tw -
#29.全球第一聯發科秀WiFi 7技術 - 經濟日報
聯發科強調,該公司已驗證其Filogic WiFi 7技術可達到IEEE 802.11be定義的最大速度,並展示多重連接模式技術(Multi-Link Operation,MLO),可同時 ... 於 money.udn.com -
#30.Performance and Coexistence Evaluation of IEEE 802.11be ...
Wi-Fi 7 is already in the making, and Multi-Link Operation (MLO) is one of the main features proposed in its correspondent IEEE 802.11be amendment. 於 www.zhuanzhi.ai -
#31.Broadcom Launches Wi-Fi 7 Portfolio for Access Points and ...
The client multi-link operation feature can keep both uplink and downlink latencies between 5 and 10ms in situations where both 5 and 6 GHz ... 於 www.anandtech.com -
#32.還沒換WiFi 6嗎?極速Wi-Fi 7世界將於2024年降臨
l 支援Multi-link Operation多重連接模式,STA能夠同時透過不同頻段或不同頻道與AP連結,進一步提升最大傳輸速度(多個頻段∕多個頻道同時傳輸)、 ... 於 onegeek1979.com -
#33.Intel闡述Wi-Fi 7無線網路技術設計方向,將比Wi-Fi 6快3.6倍
... 通訊頻道情況,則是增加Multi-RU功能,可避開因在地法規無法使用頻率,並且透過其他頻率傳輸資料,另外也支援Multi-link Operation多重連接模式, ... 於 m.eprice.com.tw -
#34.IEEE802.11be多鏈路操作系統上的平均數據封包和重排序延遲 ...
詳目顯示 ; Evaluations of the Average Packet and Resequencing Latency on IEEE802.11be Multi-Link Operation Systems · 林永松 · 碩士 · 國立臺灣大學 · 資訊管理組. 於 ndltd.ncl.edu.tw -
#35.全球第一快!聯發科率先端WiFi 7 實作技術
聯發科強調,該公司已驗證其Filogic WiFi 7 技術可達到IEEE 802.11be 定義的最大速度,並展示多重連接模式技術(Multi-Link Operation,MLO),可同時 ... 於 www.inside.com.tw -
#36.First live demo of WiFi 7 technology - eeNews Europe
The live demos shows how its Filogic chip can achieve the maximum speed defined by IEEE 802.11be and demonstrates its multi-link operation ... 於 www.eenewseurope.com -
#37.Wi-Fi 7: The future of Wi-Fi and what it will bring to the table
... the wireless technology began appearing in several new products. ... shared in a video that Wi-Fi 7 will use Multi-Link Operation (MLO). 於 www.pocnetwork.net -
#38.What is Wi-Fi 7? - everything RF
Multi -Link Operation enables link aggregation at the MAC layer with a link mapped to a channel and band. It provides higher throughput, lower ... 於 www.everythingrf.com -
#39.「6」還搞不定...Wi-Fi 7就快來啦!
多鏈路(multi-link)運作在Wi-Fi 7標準中預期會是強制性的,這能讓裝置橫跨不同通道或頻段同步接收/傳輸資料,資料與控制面(planes)是分開的。 於 www.eettaiwan.com -
#40.聯發科Wi-Fi 7領先亮相終端產品2023年上市 - 自由財經
聯發科表示,公司技術驗證其Filogic Wi-Fi 7技術可達到IEEE 802.11be定義的最大速度。公司指出,同時還展示了多重連接模式技術(Multi-Link Operation, ... 於 ec.ltn.com.tw -
#41.Reliable and Available Wireless Technologies - IETF
The multi-link operation (MLO) has been already introduced in the 802.be Draft and it can also enhance latency and reliability by enabling ... 於 www.ietf.org -
#42.全球第一Wi-Fi 7來了!聯發科技現場展示技術 - JazzLife
聯發科技驗證了其Filogic Wi-Fi 7技術可達到IEEE 802.11be定義的最大速度。同時,該公司還展示了多重連接模式技術(Multi-Link Operation,MLO),可同時 ... 於 jazznews.com.tw -
#43.Intel 解構下世代Wi-Fi 7 技術!速度可達30Gbps!
Multi -link Operation 同時也有效提升可靠性及降低延遲。最後,Wi-Fi 7 更會同時橫跨2.4GHz、5GHz、6GHz、60GHz 頻段,並引入強化版Wi-Fi 感知( ... 於 ezone.ulifestyle.com.hk -
#44.Wi-Fi 7: The Next Generation - Understanding The Future of ...
Multi -link Operation (MLO) enables devices to simultaneously transmit and receive across different bands and channels. Wi-Fi 7 seeks to enhance ... 於 www.versatek.com -
#45.Broadcom launches Wi-Fi 7 chips - Embedded.com
A feature of Wi-Fi 7 is advanced multi-link operation (MLO). This allows devices to aggregate channels and to rapidly switch between ... 於 www.embedded.com -
#46.Qualcomm Introduces World's First Wi-Fi 7 Solution
Claimed to be the world's first Wi-Fi 7 commercial solution, the new FastConnect 7800 chip supports Wi-Fi 7 multi-link operations including ... 於 www.lowyat.net -
#47.Workshop on ns-3 2020 - IEEE 802.11be Multi-link Operation ...
Let's take a closer look at how the architecture would look like. We refer to 802.11 device capable of MLO as a Multi-link Device (MLD). MLD is ... 於 sharan-naribole.github.io -
#48.Understanding IEEE 802.11be Wi-Fi 7 - Electronics Notes
Multi -Link Operation, MLO enables the devices known as Multi-Link Devices, or MLDs to use multiple channels at one. The technique also uses load balancing ... 於 www.electronics-notes.com -
#49.IEEE 802.11be Multi-Link Operation: When the Best Could Be ...
Abstract: The multi-link operation (MLO) is a new feature proposed to be part of the IEEE 802.11be Extremely High Throughput (EHT) amendment. 於 ieeexplore.ieee.org -
#50.Intel 正醞釀下一代Wi-Fi 7 無線網路連結未來
Wi-Fi 7 還支援Multi-link Operation 多重連接模式,允許裝置可以在多天線串流下,連接不同的不同頻段或不同頻道與AP 連結,進一步提升最大傳輸速度( ... 於 news.xfastest.com -
#51.Key Advantages of Wi-Fi 7: - cloudfront.net
Multi -Link Operation (MLO) is an aggregation of multiple bands or channels. With MLO,. Multi-Link Devices (MLDs) can simultaneously use the 2.4GHz, 5GHz and ... 於 d86o2zu8ugzlg.cloudfront.net -
#52.聯發科秀WiFi 7技術終端產品2023年上市 - Yahoo奇摩新聞
聯發科表示,公司技術驗證其Filogic Wi-Fi 7技術可達到IEEE 802.11be定義的最大速度。同時展示了多重連接模式技術(Multi-Link Operation,MLO),可同時 ... 於 tw.tech.yahoo.com -
#53.Wi-Fi 7 - Mpirical
That said, the IEEE have already proposed several features for Wi-Fi 7 (Release 1 / Release 2) which ... Figure 3 Multi-link Operation ... 於 www.mpirical.com -
#54.ieee p802.11-task group be (eht) meeting update
o PHY preamble design, SIG field(s) content, 16 spatial stream support, multi-link operation, power save,. o Low latency/prioritized access, preamble puncturing ... 於 www.ieee802.org -
#55.IEEE 802.11be多链路操作:最好的情况是仅使用单个接口
The multi-link operation (MLO) is a new feature proposed to be part of the IEEE 802.11be Extremely High Throughput (EHT) amendment. 於 www.x-mol.com -
#56.Time-Sensitive Networking in IEEE 802.11be - arXiv Vanity
1: Multi-link aggregation and operation techniques at ISM 2.4, 5, and 6 GHz frequency bands. Note that number, distribution and width of channels are only ... 於 www.arxiv-vanity.com -
#57.LitePoint's Latest WiFi 7 Test Solution
... 16 spatial streams and multi-link operation (MLO) are pushing the limits of Wi-Fi device's RF performance and are ushering in a new era in connectivity. 於 www.meffert-mt.de -
#58.BCM43740 & BCM43720 - Broadcom Inc.
nel Link Operation. • Multi-channel link support makes real-time decisions to provide ... high-bandwidth concurrent multi- user data transfer. Overview. 於 docs.broadcom.com -
#59.Wi-Fi 7 is less than three years away and up to five times ...
Above: Data rates for Wi-Fi 7 (left) & the concept of Multi-Link Operation (MLO, right). Graphic courtesy Intel. By Claus Hetting, Wi-Fi NOW ... 於 wifinowglobal.com -
#60.What is high-band multi-link for Wi-Fi 7? - RCR Wireless News
Multi -link capability is one of the core aspects of Wi-Fi 7, according to Qualcomm's VP and GM of Mobile and Compute Connectivity. 於 rcrwireless.com -
#61.Can Wi-Fi 7 Support Real-Time Applications? On the Impact of ...
Abstract—Multi Link Aggregation (MLA) is a feature likely to be introduced in Wi-Fi 7, ... operating in multiple bands such as the 2.4 GHz band and. 於 winser.ece.vt.edu -
#62.Wi-Fi 7箭在弦上LitePoint大秀旗艦級測試解決方案 - Digitimes
Chow的簡報著重於幾個主要功能,首先是多鏈路操作MLO (Multi-Link Operation)規格的進展,由於Wi-Fi 7的使用情境看準了虛擬實境AR/VR與元宇宙的應用, ... 於 www.digitimes.com.tw -
#63.What Is WiFi 7? WiFi 7 vs. WiFi 6 - Huawei Technical Support
Based on Wi-Fi 6, Wi-Fi 7 introduces technologies such as 320 MHz bandwidth, 4096-quadrature amplitude modulation (QAM), multi-resource unit (RU), multi-link ... 於 info.support.huawei.com -
#64.Wi-Fi 7, Data Rates, and Latency: Understanding the IEEE ...
In multi-link operation, multiple client stations with their own links function collectively as “multi-link devices” that have one interface ... 於 www.allaboutcircuits.com -
#65.聯發科秀WiFi 7技術終端產品2023年上市 - 工商時報
聯發科技驗證了其Filogic Wi-Fi 7技術可達到IEEE 802.11be定義的最大速度。同時,該公司還展示了多重連接模式技術(Multi-Link Operation,MLO),可同時 ... 於 ctee.com.tw -
#66.[2201.07499] Multi-link Operation in IEEE 802.11be WLANs
由 Á López-Raventós 著作 · 2022 · 被引用 3 次 — Abstract: The multi-link operation (MLO) is a new feature proposed to be part of the IEEE 802.11be Extremely High Throughput (EHT) amendment ... 於 arxiv.org -
#67.聯發科搶先完成WiFi 7技術展示終端產品將於2023上市
... 802.11be 定義的最大速度,同時還展示多重連接模式技術(Multi-Link Operation,MLO),能同時聚合不同頻段上的多個頻道,即使某些頻段受到干擾或 ... 於 today.line.me -
#68.access point and station multi-link device operation
Various embodiments relate to a method performed by a multi-link device (MLD) access point to establish a target wake time (TWT) with a MLD ... 於 patents.justia.com -
#69.Wi-Fi 7 and Beyond - Intel
Multi -link operation. Multi-AP operation. Deterministic low latency. Multi-RU (puncturing). * Accurate as of June/2020. Feature set and their specification ... 於 www.intel.com -
#70.What is WiFi 7? An in-depth overview of the future WiFi - TP-Link
Building off WiFi 6, WiFi 7 introduces 320 MHz ultra-wide bandwidth, 4096-QAM, Multi-RU, and Multi-Link operation. Why we need WiFi 7? 於 www.tp-link.com -
#71.A Study on Multi-link Channel Access for IEEE802.11be - IEICE
In IEEE802.11be, multi-link operation in which one terminal operates multiple frequency bands in parallel, is being considered. In order to use ... 於 www.ieice.org -
#72.全球第一聯發科技現場展示Wi-Fi 7技術 - 中時新聞網
聯發科驗證了其Filogic Wi-Fi 7技術可達到IEEE 802.11be定義的最大速度。同時,該公司還展示了多重連接模式技術(Multi-Link Operation,MLO), ... 於 www.chinatimes.com -
#73.MediaTek says Wi-Fi 7 can replace Ethernet • The Register
... speed defined by IEEE 802.11be, and said that its demonstration shows off the multi-link operation (MLO) technology in operation. 於 www.theregister.com -
#74.Understanding Link and Multilink Services | Junos OS
Multilink-based protocols enable you to split, recombine, and sequence datagrams across multiple logical data links. The goal of a multilink operation is to ... 於 www.juniper.net -
#75.Performance Analysis of Synchronous Multi-Radio ... - MDPI
The multi-link operation discussed in IEEE 802.11be allows multi-link devices to establish multiple links and operate them simultaneously. Since ... 於 mdpi-res.com -
#76.聯發科插旗Wi-Fi 7 引爆WLAN卡位戰火 - 新電子
MTK驗證了其Filogic Wi-Fi 7技術可達到IEEE 802.11be定義的最大速度。同時,該公司還展示了多重連接模式技術(Multi-Link Operation, MLO),可同時聚合不同 ... 於 www.mem.com.tw -
#77.MLO: A Big Deal When Wi-Fi 7 Arrives - Tonex Training
But wider channels and faster modulation may not even be the most important aspects of the new standard. Wi-Fi 7 will introduce the concept of Multi-Link ... 於 www.tonex.com -
#78.[產業新聞] 全球第一!聯發科技現場展示Wi-Fi 7 技術
聯發科技驗證了其Filogic Wi-Fi 7 技術可達到IEEE 802.11be 定義的最大速度。同時,該公司還展示了多重連接模式技術(Multi-Link Operation,MLO) ... 於 www.twiota.org -
#79.Wi-Fi 7 要來了!! 加入大量新技術速度提升至30Gbps 預計2024 ...
支援Multi-link Operation多重連接模式,STA能夠同時透過不同頻段或不同頻道與AP連結,進一步提升最大傳輸速度(多個頻段∕多個頻道同時傳輸)、可靠 ... 於 www.hkepc.com -
#80.CTS236: 802.11be - The Future of Wi-Fi - Clear To Send
4096 QAM; 320 MHz wide channels; MU-MIMO-16 spatial stream; Time-sensitive networking; Multi-link operation; Implicit sounding ... 於 www.cleartosend.net -
#81.Overview of multi-link operations. | Download Scientific Diagram
Multi -link operation is a new feature of IEEE 802.11be Extremely High Throughput (EHT) that enables the utilization of multiple links using individual ... 於 www.researchgate.net -
#82.Performance Analysis of Synchronous Multi-Radio ... - ProQuest
The multi-link operation discussed in IEEE 802.11be allows multi-link devices to establish multiple links and operate them simultaneously. Since the medium ... 於 search.proquest.com -
#83.聯發科首秀Wi-Fi 7:比Wi-Fi 6快2.4倍,峰值速度可到30Gbps
據悉,現場展示中,聯發科Wi-Fi 7 Filogic技術產品達成了IEE 802.11be規範定義的理論最大速度,同時MLO(multi-link operation)多重連接模式技術,可 ... 於 www.techbang.com -
#84.全球第一!聯發科技Filogic平台展示Wi-Fi 7技術
聯發科技驗證了其Filogic Wi-Fi 7技術可達到IEEE 802.11be定義的最大速度。同時,該公司還展示了多重連接模式技術(Multi-Link Operation,MLO),可 ... 於 www.stufftaiwan.com -
#85.An Experimental Study of Latency for IEEE 802.11be Multi-link ...
Abstract—Will Multi-Link Operation (MLO) be able to improve the latency of Wi-Fi networks? MLO is one of the most disruptive. MAC-layer techniques included ... 於 networks.rice.edu -
#86.查看论文信息
Research On 802.11be Enhanced Multi-Link Transmission Technology ... 802.11be proposes an enhanced multi-link single radio operation, when a terminal device ... 於 202.117.122.53 -
#87.Wi-Fi 7 - LitePoint
With MLO, 802.11be Multi-Link Devices (MLD) have the ability to support Simultaneous Transmit Receive operation (STR) by enabling simultaneous uplink and ... 於 www.litepoint.com -
#88.#Multi Link Operation | NewsBreak
#Multi Link Operation. No related news yet. Terms of UsePrivacy PolicyDo Not Sell My InfoHelp CenterAbout. © 2022 Particle Media. Get the app. 於 www.newsbreak.com -
#89.Multilink Procedure - Wikipedia
The MultiLink Procedure (MLP) (Subscription-time selectable option) exists as an added upper sublayer of the Data Link Layer, operating between the Packet ... 於 en.wikipedia.org -
#90.MULTI-LINK OPERATION FOR A SINGLE RADIO MULTI-LINK ...
This disclosure describes systems, methods, and devices related to multi-link operation. A device may configure a single N×N transmit ... 於 www.freepatentsonline.com -
#91.WiFi 7(802.11 be)相关知识汇总
... MLO(Multi-Link Operation)和Multi-RU(Preamble Puncturing),允许不同的频带、信道间进行链路层面上的聚合和协作;在多频带/信道下进行流量切换(traffic ... 於 wirelesslink.net -
#92.802.11be testing Wi-Fi 7 testing | Rohde & Schwarz
... units (RU) per user, optimized HARQ process, multi-link operation over all bands, and several kinds of coordinated multi access point operations. 於 www.rohde-schwarz.com -
#93.Let me know the multi-link. | FAQs | Proface - It works
For information on Multilink, refer to the following. ... display units increases, the display and operation speeds on the display units become slower. 於 origin-faq.pro-face.com -
#94.Intel展望未來技術,分享預計2024年才會商用化的Wi-Fi 7技術 ...
3、加入對Multi-Link Operation多重連線模式藉此結合不同頻段、不同頻道與AP提升傳輸速度與降低延遲。 4、路由器可保留排外的特殊傳輸時段應對需要 ... 於 inewsdb.com -
#95.Multi-Link Operation with Enhanced Synchronous Channel ...
Multi -link operation (MLO) is the concurrent utilization of multiple radio links of different frequency channels/bands by an AP, a client, or ... 於 www.ncbi.nlm.nih.gov -
#96.IEEE802.11be多鏈路操作系統上的平均數據封包和重排序延遲 ...
Evaluations of the Average Packet and Resequencing Latency on IEEE802.11be Multi-Link Operation Systems. 徐孟祺 , 碩士指導教授:林永松. 於 www.airitilibrary.com -
#97.Broadcom Launches Wi-Fi 7 Chips - EE Times Asia
A feature of Wi-Fi 7 is advanced multi-link operation (MLO). This allows devices to aggregate channels and to rapidly switch between ... 於 www.eetasia.com