Git learning的問題,透過圖書和論文來找解法和答案更準確安心。 我們找到下列懶人包和總整理
Git learning的問題,我們搜遍了碩博士論文和台灣出版的書籍,推薦寫的 Mastering Git: A Beginner’’s Guide 和的 Mastering Git: A Beginner’’s Guide都 可以從中找到所需的評價。
這兩本書分別來自 和所出版 。
國立政治大學 資訊科學系 紀明德所指導 李宣毅的 無人機基於深度強化學習於虛擬環境之視覺化分析 (2021),提出Git learning關鍵因素是什麼,來自於深度強化學習、無人機競賽、虛擬環境、視覺化分析。
而第二篇論文長庚大學 電機工程學系 沙庫瑪所指導 Djeane Debora Onthoni的 使用深度學習方法分析ADPKD患者的非顯影和顯影電腦斷層圖像的電腦視覺任務 (2021),提出因為有 no的重點而找出了 Git learning的解答。
Mastering Git: A Beginner’’s Guide
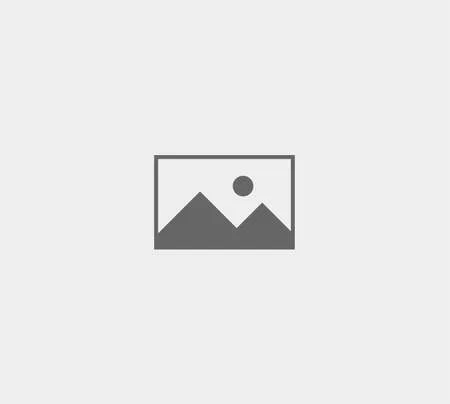
為了解決Git learning 的問題,作者 這樣論述:
Sufyan bin Uzayr is a writer, coder and entrepreneur with over a decade of experience in the industry. He has authored several books in the past, pertaining to a diverse range of topics, ranging from History to Computers/IT.Sufyan is the Director of Parakozm, a multinational IT company specializing
in EdTech solutions. He also runs Zeba Academy, an online learning and teaching vertical with a focus on STEM fields.Sufyan specializes in a wide variety of technologies, such as JavaScript, Dart, WordPress, Drupal, Linux and Python. He holds multiple degrees, including ones in Management, IT, Liter
ature and Political Science.Sufyan is a digital nomad, dividing his time between four countries. He has lived and taught in universities and educational institutions around the globe. Sufyan takes a keen interest in technology, politics, literature, history and sports, and in his spare time, he enjo
ys teaching coding and English to young students.Learn more at sufyanism.com
Git learning進入發燒排行的影片
แชร์โปรแกรมสำหรับถึงข้อมูล COVID-19 แยกตามวันในแต่ละประเทศ
วิธีการใช้ Visual Studio Code ร่วมกับ github.com เพื่อแชร์โค้ดและข้อมูล COVID-19
github ► https://github.com/prasertcbs/covid19
เชิญสมัครเป็นสมาชิกของช่องนี้ได้ที่ ► https://www.youtube.com/subscription_center?add_user=prasertcbs
สอน data science ด้วยข้อมูล COVID-19 ► https://www.youtube.com/watch?v=yEiBeyEASRM&list=PLoTScYm9O0GEZpIlV6-_ppgQmBO0luWG-
สอน Pandas ► https://www.youtube.com/playlist?list=PLoTScYm9O0GGsOHPCeufxCLt-uGU5Rsuj
สอน git ► https://www.youtube.com/playlist?list=PLoTScYm9O0GGsV1ZAyP4m_iyAbflQrKrX
สอน visual studio code ► https://www.youtube.com/playlist?list=PLoTScYm9O0GEo8pnhJb-m-MGVGDvGb4bB
สอน Jupyter Notebook ► https://www.youtube.com/playlist?list=PLoTScYm9O0GErrygsfQtDtBT4CloRkiDx
สอน Machine learning ► https://www.youtube.com/playlist?list=PLoTScYm9O0GH_3VrwwnQafwWQ6ibKnEtU
สอน Python สำหรับ data science ► https://www.youtube.com/playlist?list=PLoTScYm9O0GFVfRk_MmZt0vQXNIi36LUz
สอน Numpy ► https://www.youtube.com/playlist?list=PLoTScYm9O0GFNEpzsCBEnkUwgAwOu_PWw
สอน matplotlib ► https://www.youtube.com/playlist?list=PLoTScYm9O0GGRvUsTmO8MQUkIuM1thTCf
สอน seaborn ► https://www.youtube.com/playlist?list=PLoTScYm9O0GGC9QvLlrQGvMYatTjnOUwR
สอนภาษาไพธอน Python เบื้องต้น ► https://www.youtube.com/playlist?list=PLoTScYm9O0GH4YQs9t4tf2RIYolHt_YwW
สอนภาษาไพธอน Python OOP ► https://www.youtube.com/playlist?list=PLoTScYm9O0GEIZzlTKPUiOqkewkWmwadW
สอน Python 3 GUI ► https://www.youtube.com/playlist?list=PLoTScYm9O0GFB1Y3cCmb9aPD5xRB1T11y
#prasertcbs_datascience #prasertcbs #prasertcbs_pandas #github
無人機基於深度強化學習於虛擬環境之視覺化分析
為了解決Git learning 的問題,作者李宣毅 這樣論述:
近年來非常流行全自動無人機競賽,2019 年微軟團隊 Airsim 於NeurlIPS 的會議上舉辦一個基於虛擬環境的無人機過框比賽,其主要目標希望能夠超越人類玩家的表現,而在得名的參賽者中並沒有針對這項競賽設計一套利用深度強化學習的方法,因此本研究針對此虛擬競賽使用深度強化學習的方法訓練成功過框完賽的模型,並結合現實中無人機時常運用的 ROS 系統作為指令傳遞的通訊架構縮小虛擬與現實的差異。眾所周知深度強化學習這項方法就如同黑盒子,使用者不知道模型究竟學習到什麼,因此本研究設計一套視覺化介面,提供使用者分析模型表現,並設計一套圖表分析各項動作選擇的機率,看出模型在當下狀態所做的思考是否與普
遍認知上相同,最後利用神經網路視覺化的技巧看出模型表現不佳的問題並將其改良,其中發現某些情況下模型表現與人類的行為相似,使得對深度強化學習的信任以及現實應用的可能性大幅增加。
Mastering Git: A Beginner’’s Guide
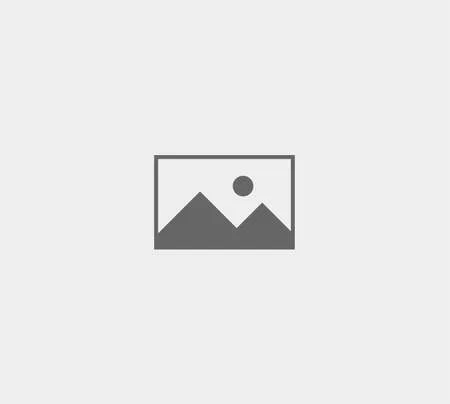
為了解決Git learning 的問題,作者 這樣論述:
Sufyan bin Uzayr is a writer, coder and entrepreneur with over a decade of experience in the industry. He has authored several books in the past, pertaining to a diverse range of topics, ranging from History to Computers/IT.Sufyan is the Director of Parakozm, a multinational IT company specializing
in EdTech solutions. He also runs Zeba Academy, an online learning and teaching vertical with a focus on STEM fields.Sufyan specializes in a wide variety of technologies, such as JavaScript, Dart, WordPress, Drupal, Linux and Python. He holds multiple degrees, including ones in Management, IT, Liter
ature and Political Science.Sufyan is a digital nomad, dividing his time between four countries. He has lived and taught in universities and educational institutions around the globe. Sufyan takes a keen interest in technology, politics, literature, history and sports, and in his spare time, he enjo
ys teaching coding and English to young students.Learn more at sufyanism.com
使用深度學習方法分析ADPKD患者的非顯影和顯影電腦斷層圖像的電腦視覺任務
為了解決Git learning 的問題,作者Djeane Debora Onthoni 這樣論述:
ContentsABSTRACT. . . . . . . iTABLE OF CONTENTS. . . . . . . iiLIST OF FIGURES. . . . . . . viLIST OF TABLES. . . . . . . viiiLIST OF ABBREVIATIONS. . . . . . . ix1 Introduction 11.1 Medical Imaging . . . . . . . . . . . . . . . . . . 11.1.1 Ultrasound . . . . . . . . . . . . . . . . . . . 11.1.2
Magnetic Resonance Imaging . . . . . . . . . . . 21.1.3 Computed Tomography . . . . . . . . . . . . . . . 31.2 Artificial Intelligence . . . . . . . . . . . . . . 51.2.1 Machine Learning . . . . . . . . . . . . . . . . 51.2.1.1 Supervised Learning . . . . . . . . . . . . . . 51.2.1.2 Unsupervised
Learning . . . . . . . . . . . . . 61.2.2 Deep Learning . . . . . . . . . . . . . . . . . . 71.2.2.1 Classification Task . . . . . . . . . . . . . . 81.2.2.2 Localization Task . . . . . . . . . . . . . . . 91.2.2.3 Segmentation Task . . . . . . . . . . . . . . . 101.3 Kidney Disease . . . . . . . .
. . . . . . . . . . 111.4 Motivations . . . . . . . . . . . . . . . . . . . . 131.4.1 Non-contrast-enhanced Computed Tomography . . . . 141.4.2 Contrast-enhanced Computed Tomography . . . . . . 151.4.3 Localization and Segmentation for analyzing TKV . 151.5 Main Contributions . . . . . . . . . . .
. . . . . 161.6 Thesis Organization . . . . . . . . . . . . . . . . 162 Related works 182.1 Without Artificial Intelligence . . . . . . . . . . 182.2 With Artificial Intelligence . . . . . . . . . . . 192.2.1 Localization of ADPKD . . . . . . . . . . . . . . 192.2.2 Segmentation of ADPKD . . . .
. . . . . . . . . . 213 Automatic ADPKD Kidneys Localization Model on NCCT and CCT 233.1 Introduction . . . . . . . . . . . . . . . . . . . 233.2 Materials and Methods . . . . . . . . . . . . . . . 243.2.1 Data Acquisition . . . . . . . . . . . . . . . . 243.2.2 Ground Truth Annotation . . . . . .
. . . . . . . 253.2.3 Methods . . . . . . . . . . . . . . . . . . . . . 253.2.3.1 Preprocessing . . . . . . . . . . . . . . . . . 253.2.3.2 Dataset Partition . . . . . . . . . . . . . . . 273.2.3.3 Bounding Box Labeling . . . . . . . . . . . . . 283.2.3.4 Automatic ADPKD Kidneys Localization Model
. . .283.2.3.5 Training and Tuning Model . . . . . . . . . . . 303.2.3.6 Image-Wise and Subject-Wise Testing and Evaluation . . 313.2.4 Experimental Setup . . . . . . . . . . . . . . .. 313.2.5 Evaluation Metrics . . . . . . . . . . . . . . . 313.2.6 Evaluation Procedures . . . . . . . . . . . . .
. 323.3 Results on NCCT and CCT . . . . . . . . . . . . . . 333.3.1 Evaluation Results of Validation set on NCCT . . 333.3.2 Evaluation Results of Testing set on NCCT . . . . 333.3.3 Evaluation Results of Validation Set on CCT . . . 343.3.4 Evaluation Results of Testing set on CCT . . . . 353.4 Ev
aluation Results of Image-Wise Testing . . . . . 363.5 Evaluation Results of Subject-Wise Testing . . . . 383.6 Discussion . . . . . . . . . . . . . . . . . . . . 403.7 Conclusion . . . . . . . . . . . . . . . . . . . . 464 Automatic ADPKD kidneys Segmentation Model and TKV Estimation Modelon NC
CT and CCT 484.1 Introduction . . . . . . . . . . . . . . . . . . . 484.2 The Proposed Method . . . . . . . . . . . . . . . . 504.2.1 Data Preprocessing . . . . . . . . . . . . . . . 504.2.2 Automatic ADPKD Kidneys Segmentation . . . . . . 514.2.3 TKV Estimation Model . . . . . . . . . . . . . .
534.3 Experiment and Results . . . . . . . . . . . . . . 544.3.1 Dataset . . . . . . . . . . . . . . . . . . . . . 544.3.2 Experimental Setup . . . . . . . . . . . . . . . 554.3.3 Evaluation Metrics . . . . . . . . . . . . . . . 564.3.4 ADPKD Kidney Segmentation Results . . . . . . . . 564.3.4.1
Validation set results on NCCT . . . . . . . . 574.3.4.2 Testing set results on NCCT . . . . . . . . . . 57vii4.3.4.3 Validation set results on CCT . . . . . . . 584.3.4.4 Testing set results on CCT . . . . . . . . . . 594.3.5 TKV Estimation Results . . . . . . . . . . . . . 604.4 Discussion .
. . . . . . . . . . . . . . . . . . . 604.5 Conclusion . . . . . . . . . . . . . . . . . . . . 645 Conclusions and Future works 655.1 Conclusions . . . . . . . . . . . . . . . . . . . . 655.2 Future Works . . . . . . . . . . . . . . . . . . . 66Bibliography 67List of Figures1.1 Types of medical i
maging. . . . . . . . . . . . . . 21.2 Supervised and Unsupervised Learning algorithms based on tasks. . . . . . 61.3 Various architectures based on computer vision tasks. . . . . . . . . . . . . 81.4 Healthy kidneys. . . . . . . . . . . . . . . . . . 121.5 ADPKD kidneys on NCCT and CCT, and renal
cyst: (a) ADPKD kidneyand liver cyst on CCT; (b) ADPKD kidney and liver cyst on NCCT; (c)ADPKD kidney, liver, and spleen; (d) Renal cyst in non-ADPKD. . . . . . 143.1 Raw image and respective ground truth images: (a) Raw image; (b) Groundtruth for right kidney (Green); (c) Ground truth for left kidn
ey (Yellow); (d)Ground truth for both right (Green) and left (Yellow) kidneys. . . . . . . . 253.2 Proposed automatic ADPKD kidneys localization model framework. . . . . 263.3 Preprocessing procedures. .. . . . . . . . . . . . 263.4 The architecture of automatic ADPKD kidneys localization model, whe
rejfj, C(x, y), w, h, and V2 refer to as total number of feature maps, centerbounding box, width, height, and version 2, respectively. . . . . . . . . . . 293.5 Precision and recall curve on NCCT: (a) Right kidney; (b) Left kidney. . . . 363.6 Automatic ADPKD kidneys localization results on NCCT. .
. . . . . . . . 363.7 Precision and recall curve on CCT: (a) Right kidney; (b) Left kidney. . . . . 393.8 Automatic ADPKD kidneys localization results on CCT. . . . . . . . . . . 393.9 Precision and recall curve of our model on image-wise testing set: (a) Rightkidney; (b) Left kidney. . . . . . . .
. . . . . . . 41xiv3.10 Comparison of classification and localization loss on image-wise testing set. 423.11 Detection results: (a) ADPKD kidneys associated with liver cysts; (b)ADPKD kidneys with adjacent organs. . . . . . . . . . 423.12 Precision and recall curve of our model on subject-wise test
ing: (a) Rightkidney; (b) Left kidney. . . . . . . . . . . . . . .. 433.13 Comparison of classification and localization loss on image-wise testing set. 443.14 Detection results: (a) Small size of ADPKD kidneys; (b) Big size of ADPKDkidneys. . . . . . . . . . . . . . . . . . . . . . .. 453.15 Miscla
ssification and mislocalization example: (a) Misclassification; (b)Mislocalization. . . . . . . . . . . . . . . . . . .. 464.1 The overview of proposed method work flow. . . .. 504.2 The overview of data preprocessing. . . . . . . . 514.3 The overview of automatic ADPKD kidneys segmentation model. .
. . . . 534.4 Automatic ADPKD kidneys segmentation ROC curve on NCCT. . . . . . . 604.5 Automatic ADPKD kidneys segmentation results on NCCT. . . . . . . . . 614.6 Automatic ADPKD kidneys segmentation ROC curve on CCT. . . . . . . . 614.7 Automatic ADPKD kidneys segmentation results on CCT. . . . .
. . . . . 624.8 Validation curve for DTR: (a) Validation score on NCCT; (b) Validationscore on CCT. . . . . . . . . . . . . . . . . . . . 63List of Tables3.1 210 CT data acquisitions from 97 ADPKD patients. . . 243.2 Localization results using validation set with k-fold on NCCT. . . . . . . . 343.3
Localization results using testing set on NCCT. . . .353.4 Localization results using validation set with k-fold on CCT. . . . . . . . . 373.5 Localization results using testing set on CCT. . . . 383.6 Evaluation metrics results on image-wise testing. . .403.7 Comparison of AP and mAP with other ar
chitectures on image-wise testing. 413.8 Evaluation metrics results of subject-wise testing. .433.9 Comparison of AP and mAP with other architectures on subject-wise testing. 444.1 Segmentation results using validation set with k-fold on NCCT. . . . . . . . 584.2 Segmentation results using testing s
et on NCCT. . . .594.3 Segmentation results using validation set with k-fold on CCT. . . . . . . . . 624.4 Segmentation results using testing set on CCT. . . . 634.5 R2 score using validation set with k-fold on NCCT and CCT. . . . . . . . . 64