Book scanner的問題,透過圖書和論文來找解法和答案更準確安心。 我們找到下列懶人包和總整理
Book scanner的問題,我們搜遍了碩博士論文和台灣出版的書籍,推薦Thomas, Adrian寫的 Invisible Light: The Remarkable Story of Radiology 和Blackburne, Livia的 The Queen’’s Council #2 Feather and Flame都 可以從中找到所需的評價。
這兩本書分別來自 和所出版 。
臺北醫學大學 國際醫學研究博士學位學程 康峻宏、黎阮國慶所指導 TRUONG NGUYEN KHANH HUNG的 運用深度學習於膝關節損傷核磁共振影像之人工智慧偵測與診斷模型 (2021),提出Book scanner關鍵因素是什麼,來自於Artificial intelligence、deep learning、machine learning、Knee MRI、ACL、meniscus。
而第二篇論文國立虎尾科技大學 資訊工程系碩士班 徐元寶所指導 葉峻華的 可執行同時室內定位與建圖任務之差速輪機器人製作 (2021),提出因為有 差速輪機器人、粒子濾波器、雷射測距儀、現場可程式化閘陣列、同時定位與建圖的重點而找出了 Book scanner的解答。
Invisible Light: The Remarkable Story of Radiology
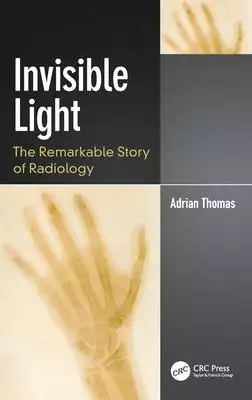
為了解決Book scanner 的問題,作者Thomas, Adrian 這樣論述:
Dr Adrian Thomas is a writer and teacher. He has seen the development of modern radiology during his career, entering medical school at University College London in 1972, the year that the CT scanner was announced. He has had an interest in history since his school days; studying medical history wit
h Jonathan Miller, Edwin Clarke and Bill Bynum at University College London for his BSc. Adrian started his radiology training in 1981 at Hammersmith Hospital, which coincided with their pioneering development of the MRI scanner. He is a founder member and past-president of the British Society for t
he History of Radiology. He has co-authored 7 books and written many book chapters. He is past-president of the British Society for the History of Medicine, past-president of the Radiology Section of the Royal Society of Medicine, is Honorary Historian to the British Institute of Radiology, and is a
visiting professor at Canterbury Christ Church University.
Book scanner進入發燒排行的影片
สั่งซื้อ Czur Aura ได้ที่ลิ้งค์นี้เลยจร้า
https://www.lazada.co.th//products/i334202530-s646980789.html?spm=a2o7g.10605368.0.0.8eef1e132sPKiW&urlFlag=true&mp=2
นี่คือเครื่องสแกนหนังสือ/เอกสาร ที่ครบเครื่องและอัจฉริยะที่สุดในช่วงเวลานี้เลยทีเดียว
ใช้งานได้ 2 โหมด ทั้งโคมไฟ และ เครื่องสแกน
โคมไฟ ปรับได้ 2 สี ความสว่างปรับได้ 6 ระดับ
เครื่องสแกนระบบอัจฉริยะ แสกนได้รวดเร็ซแม่นยำการจัดการหลังสแกนที่ยืนหยุ่นและสมบูรณ์แบบ
เรามาแกะกล่อง ประกอบ และทดลองใช้กันเลยจร้า!!!
????????????
#เพื่อนซี้ตัวแสบ #เครื่องสแกนหนังสือ #รีวิของเจ๋งๆ
#Czur #gadget
♡ Facebook ส่วนตัว : https://www.facebook.com/zeejhk
♣ Fanpage 1 : https://www.facebook.com/555infinity/
♣ Fanpage 2 : https://www.facebook.com/ZabbFamily
♤ Instagram : https://www.instagram.com/555_infinity/
♦ สำหรับส่งIntro : [email protected]
♧ Tiktok : ครอบครัวตัวแสบ
***
☞ ติดต่องาน : [email protected]
運用深度學習於膝關節損傷核磁共振影像之人工智慧偵測與診斷模型
為了解決Book scanner 的問題,作者TRUONG NGUYEN KHANH HUNG 這樣論述:
Introduction: Efficient and accurate detection is vital for the diagnosis and treatment of knee injuries. In recent years, there is an increase in interest in deep learning (DL) approaches to detecting knee injuries in magnetic resonance imaging (MRI). Studies have shown that DL models are capable
of reaching the same level as human radiologists when it comes to sensitivity and specificity, while at the same time requiring significantly less training time. Current Artificial Intelligent (AI) - based systems are, however, still limited by many different factors, such as unbalanced classes in t
raining data, or the nature of these systems which makes false positives and false negatives almost an inevitability. There are multiple routes for improving upon the existing DL knee injury detection models. As they continue to become more and more advanced, it is expected that the use of these sys
tems will become more popular in the future.Method: In this study, we create multi models based on machine learning (ML) and DL algorithms to perform classification, recognition, and segmentation tasks on knee MRI. In which the two most important components in the knee joint in this study are the an
terior cruciate ligament (ACL) and meniscus.The first model, based on the DenseNet 121 neural network structure, was used to classify images with or without ACL injury. The dataset includes 799 knee MRI reports from Cho Ray Hospital (Vietnam). These MRI data were obtained from previous work in the h
ospital, containing knee MRI reports from 5 years (January 1st, 2015 – December 31st, 2019)Using the Faster-region convolutional neural network (Faster - RCNN) and several convolutional neural networks (CNN) backbone tests, such as VGG-16, Res-Net50, DenseNet-121, EfficientNet-B0, and EfficientNetV2
- B0 algorithms, the second group of models can recognize the ACL on knee MRI as a function of the typical imaging characteristics. This research collected 256 knee MRI examinations performed at Cho Ray Hospital, Ho Chi Minh City, Vietnam, between January 1, 2018, and December 31, 2020 (including t
raining and testing datasets).The third model focuses on automatic identification and classification of meniscus based on the Yolo-v4 object detection model. At the same time, the lesion location is also shown on images by the GRAD-CAM technique. The total number of subjects used in this study was 7
04 patients, including meniscus lesions and the control group. All MRIs in this study were collected before the surgery, and all had no prior surgical history. The MRI scanner at Cho Ray Hospital is MAGNETOM Skyra 3T (Siemen), and at Hoan My Hospital is 3.0T MRI Scanners SIGNA (GE Healthcare). In ad
dition, we also used a public dataset - MRNet dataset (validation dataset) from Stanford University Medical Center with 120 examinations for external testing.Results: The area under the ROC curve (AUC) for the ACL injury classification system was 80.63% with the axial plane and around 78% with both
the sagittal and coronal planes, respectively. All sensitivity and specificity point estimates of the proposed ACL injury detection system were all over 96%, indicating no significant differences in diagnostic performance between different planes.Our DL model detected meniscus tears with 91.4% accur
acy on the internal testing dataset, 89.2% accuracy on the external validation dataset, and 79.9% accuracy on the MRNet dataset, respectively. The meniscus tears were visualized by auto-enlarging the detection area and Grad-CAM images.Conclusion: This report describes the various approaches in knee
MRI experiments to provide different AI models for the prediction of knee injuries. The CNN model applied to classify injured ACL images had high sensitivity and specificity, showing that using a simple structured 2D-CNN is more effective for small datasets and can assist non-experts in assessing th
e assessment of ACL injuries. The proposed model applied to detect meniscus lesions had high accuracy and specificity, showing that our model can assist non-experts in assessing the assessment of meniscus injuries.
The Queen’’s Council #2 Feather and Flame
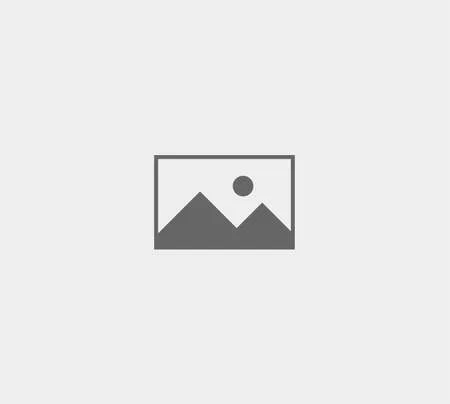
為了解決Book scanner 的問題,作者Blackburne, Livia 這樣論述:
New York Times bestselling author Livia Blackburne wrote her first novel while researching the neuroscience of reading at the Massachusetts Institute of Technology. Since then, she’s switched to full time writing, which also involves getting into people’s heads but without the help of a three tesla
MRI scanner. She is the author of the Midnight Thief, and Rosemarked series, as well as the picture book I Dream of Popo.
可執行同時室內定位與建圖任務之差速輪機器人製作
為了解決Book scanner 的問題,作者葉峻華 這樣論述:
本論文旨在實作一差速輪機器人,並以粒子濾波器(Particle Filter, PF)演算法,結合里程計和雷射測距儀(Laser Range Finder, LRF),控制所完成之機器人執行室內同時定位與建圖(Simultaneous Localization And Mapping, SLAM)任務。本研究所製作之差速輪機器人以現場可程式化閘陣列(Field Programmable Gate Array, FPGA)為底層硬體平台,負責接收來自機器人作業系統(Robot Operating System, ROS)之脈衝寬度調變(Pulse Width Modulation, PWM)控
制訊號,並驅動馬達;以及接收馬達編碼器之脈衝訊號,並傳送至機器人作業系統(Robot Operating System, ROS),作為計算機器人位姿之里程計資訊。此外,本研究在底層以PID控制法則控制馬達之運轉,以確保馬達能準確達到控制命令所要求轉速。模擬以及實驗結果顯示,本文所完成之差速輪機器人在室內環境移動,到達目標之位置誤差平均在0.15公尺內,角度誤差在10度內。